Журнал высшей нервной деятельности им. И.П. Павлова, 2021, T. 71, № 4, стр. 547-562
Alpha-Band Functional Connectivity During Modality-Specific Anticipatory Attention in Children Aged 9–10 Years: Eeg-Source Coherence Analysis
I. V. Talalay a, *, A. V. Kurgansky a, R. I. Machinskaya a
a Institute of Developmental Physiology, Russian Academy of Education
Moscow, Russia
* E-mail: etalalay.et@gmail.com
Поступила в редакцию 26.11.2020
После доработки 20.01.2021
Принята к публикации 2.03.2021
Аннотация
Functional connectivity was studied in a group of 17 right-handed children aged 9.789 ± 0.447 years during the deployment of cued anticipatory attention. Participants performed visual and auditory versions of the temporal order judgment task. Prestimulus functional links were assessed via alpha-band coherence computed in the source space for preselected regions of interest. As compared with the baseline condition, an increase of local functional links between the primary visual cortex and the intraparietal cortex was observed in both hemispheres during the anticipation of visual and auditory stimuli. An increase of functional interaction between the intraparietal cortex and the ventral premotor cortex was observed only in the left hemisphere during auditory anticipatory attention. Unlike our previous research on anticipatory attention in adults, the analysis of functional connectivity in children showed no frontoparietal functional links in the right hemisphere and no modality-specific cortical links. The results of the study suggest that the brain’s top-down modulatory systems of the right hemisphere are still immature in children aged 9–10 years.
INTRODUCTION
The anticipation of different events is incorporated into the lives of all people. It seems that all fields of human activities rest on the faculty of anticipating the future at the level of both simple actions and complex behaviors. In cognitive neuroscience, this faculty is usually called anticipatory attention (see, e.g., Näätänen, 1992; Bastiaansen, Brunia, 2001; Brunia, van Boxtel, 2004; Brunia et al., 2011; Klimesch, 2012). Anticipatory attention is directed by explicit (see, e.g., Spence, Driver, 1997; Brunia, van Boxtel, 2004; Mozolic et al., 2008; Posner, Fan, 2008; Rohenkohl et al., 2014; Talalay et al., 2018) or implicit (Cleeremans et al., 1998; Turk-Browne et al., 2010; Dale et al., 2012; Zhao et al., 2013; Altamura et al., 2014; Talalay et al., 2018) experience towards an upcoming stimulus in order to facilitate its processing (Bastiaansen, Brunia, 2001). Taking into consideration an important role anticipation plays in any goal-directed behavior, researchers try to reveal neurophysiological bases and behavioral outcomes of this cognitive function.
Basically, psychological and psychophysiological studies of anticipatory attention are conducted with the help of the Posner paradigm (Posner et al., 1980). In this paradigm, there is either central, symbolic (also referred to as “endogenous”) or peripheral (also referred to as “exogenous”) cue-stimulus, which informs participants about certain characteristics of an upcoming trial. Furthermore, the cues can contain true information (valid cueing), no information (neutral cueing) or false information (invalid cueing) about the target stimulus. This experimental model has different modifications and is mainly used to study cued endogenous (voluntary) (Folk, Hoyer, 1992; Langley et al., 2011) or exogenous (involuntary) (Akhtar, Enns, 1989; Folk, Hoyer, 1992; Perchet, Garcia-Larrea, 2000; Langley et al., 2011) attention orienting. The Posner cueing task has been embedded by J. Fan and colleagues (Fan et al., 2002) in the Attention Network Task (ANT), which is designed to measure the efficiency of the alerting, orienting and executive attention networks (Posner, Petersen, 1990; revised by Petersen, Posner, 2012; Posner, Fan, 2008). This task includes two types of cues. The first type provides information on the time of target occurrence, thus underlying the development of phasic alertness in participants. The second type is exogenous and provides information on the place of target occurrence, thus leading to changes in the orienting network. If the cue is found invalid, the disengagement and reorienting of attention take place. In the ANT, cueing is combined with the flanker task (Eriksen, Eriksen, 1974), which serves as the measure of executive attention. According to the definition of anticipatory attention given at the beginning of this article, we consider that cue-induced alerting and orienting should be regarded as anticipation-related processes.
Both the Posner cueing task (Akhtar, Enns, 1989; Folk, Hoyer, 1992; Perchet, Garcia-Larrea, 2000; Langley et al., 2011) and the ANT (Mezzacappa, 2004; Rueda et al., 2004; Konrad et al., 2005; Jennings et al., 2007; Hahn et al., 2011; Zhou et al., 2011; Williams et al., 2016; Santhana Gopalan et al., 2019) are commonly used to study age-related difference in cued attention. Data generally show a positive influence of valid cueing on the efficiency of task performance in children (Swanson et al., 1991; Mezzacappa, 2004; Rueda et al., 2004; Santhana Gopalan et al., 2019) and adults (Akhtar, Enns, 1989; Folk, Hoyer, 1992; Langley et al., 2011; Rueda et al., 2004).
A review of multiple studies on age-related changes in attention (Posner et al., 2013) suggests that there is no age difference in the orienting benefit effect (i.e., the difference in reaction time (RT) between neutral vs. valid trials) among children aged 5–6, 8–10 years and adults (Enns, Brodeur, 1989). At the same time, the speed (i.e., the difference in performance on valid trials with short vs. long cue-to-target intervals) of orienting, the ability to disengage and reorient attention voluntarily improve with age (Schul et al., 2003). In addition, there seems to be an age-related decrease in the orienting cost (i.e., the difference in RT between invalid vs. neutral trials) (Enns, Brodeur, 1989; Wainwright, Bryson, 2002; Schul et al., 2003). According to M. Corbetta and G.L. Shulman (2002), it seems that endogenous orientation (or reorientation) of attention is related to the activity of the superior parietal lobule (SPL) and the frontal eye fields (FEF), whereas exogenous orienting is linked to the activity of the temporoparietal junction (TPJ) and the ventral frontal cortex (VFC), largely lateralized to the right hemisphere. A large number of studies emphasize the role of the dorsal frontoparietal network (including the intraparietal sulcus [IPS] and the FEF) in the mediation of top-down control of spatial cued attention (Capotosto et al., 2009, 2012; Simpson et al., 2011; Shomstein, 2012; Liu et al., 2016).
Concerning the alerting network, the presentation of a warning cue develops the state of alertness in participants, thus making them anticipate an upcoming trial and respond faster (Posner et al., 2013, review). However, short cue-to-target intervals may also cause declines in performance accuracy (Posner, 1978). The efficiency of task performance was found to improve with age (Rueda et al., 2004; Mezzacappa, 2004; Morrison, 1982). The developmental changes in alertness during childhood might be related to continuous maturation of frontal systems during this period (Posner et al., 2013, review). In (Santhana Gopalan et al., 2019), the analysis of brain event-related potentials (ERP) and their source localization showed that both the alerting and orienting networks were associated with a low level of frontal and parietal activation in children aged 12–13 years. At the same time, the adult attention network seems to bear on frontal and parietal areas to maintain alertness (Fan et al., 2005; Périn et al., 2010). P. Santhana Gopalan and colleagues suggest that this maintenance of alertness and readiness matures only in late childhood (after 12 years of age). According to the authors, frontoparietal activity in adults might reflect more top-down control of attention that is not utilized by children during the performance of the ANT (Casey et al., 2004).
Many studies of the brain’s mechanisms underlying alertness are based on the analysis of the contingent negative variation (CNV) (Walter, 1964). The CNV is a slow negative wave observed at central and frontocentral sites along the midline in the interval between a warning signal and an upcoming target (Williams et al., 2016). This pattern of brain-generated electrical activity appears to index anticipatory attention, motivation, and motor preparation (Tecce, 1972; Ulrich et al., 1998; Leuthold, Jentzsch, 2001; Guo et al., 2019). It has been identified that the prefrontal cortex (PFC; Rosahl, Knight, 1995), the anterior cingulate cortex (ACC) (Gómez et al., 2003; Segalowitz, Davies, 2004; Fan et al., 2007), the basal ganglia (BG) (Bares, Rektor, 2001), and the supplementary motor area (SMA) (Gómez et al., 2003) are involved in the generation of the CNV. The frontal early CNV component is considered to reflect an orienting response and the central late CNV component is described as a motor preparation (see, e.g., Gómez et al., 2003). The amplitude of the CNV was shown to increase with age, especially during middle childhood (Jonkman, 2006). In comparison with adults, children display smaller early CNV amplitudes at frontocentral locations (Jonkman et al., 2003), suggesting a role of frontal lobe maturation in the alerting network.
Other popular electrophysiological indices of anticipatory processes in the brain are the stimulus-preceding negativity (SPN) (see, e.g., Brunia, van Boxtel, 2004) and the Bereitschaftspotential (BP) (see, e.g., Shibasaki, Hallett, 2006; Di Russo et al., 2017; Bianco et al., 2020). Both indices are commonly used to study the selectivity of anticipatory processes in the brain. The BP is a measure of activity in the motor cortex and the supplementary motor area that precedes any voluntary motor act. The SPN is observed over frontal areas and reflects perceptual anticipation. C.H.M. Brunia and G.J.M. van Boxtel (2004) used this index to study anticipatory attention to verbal and non-verbal stimuli. They employed the time estimation paradigm along with a block experimental design (Brunia, Damen, 1988; Damen, Brunia, 1987). Adult participants were asked to respond within a certain time window after the presentation of an imperative signal. After that, auditory and visual stimuli (referred to as “knowledge-of-results” [KR] stimuli) were presented in verbal and nonverbal modes informing participants about the correctness of their response. The order of KR stimuli within a block was set for the cause of modality-specific anticipation of these stimuli. The analysis of ERP parameters showed that anticipatory attention to visual and auditory KR stimuli was accompanied by an increase of local activity in modality-specific cortical areas as reflected in the SPN and event-related desynchronization of alpha-band oscillations (Bastiaansen et al., 2001). These data are concordant with the results of some studies indicating modality specificity of anticipatory attention (Spence, Driver, 1997; Machinskaya, 1998; Mozolic et al., 2008). C. Spence and J. Driver (1997) showed that the presentation of a symbolic visual cue predicting the likely target modality (visual or auditory) improved performance accuracy and reduced RT in the case of expected vs. unexpected stimulus modality. J.L. Mozolic and colleagues (Mozolic et al., 2008) conducted an fMRI study of cued modality-specific attention. According to that study, the activity of visual cortices in the prestimulus period is higher for visual selective attention than for auditory selective attention, and vice versa. Furthermore, the results of an EEG study of functional connectivity during selective anticipatory attention (Machinskaya, 1998) showed modality-specific functional cortical links during the anticipation of auditory and tactile stimuli. S.M. Weiss and colleagues (Weiss et al., 2018) studied tactile anticipatory attention in children aged 6–8 years. They measured electroencephalographic activity over the sensorimotor cortex after the presentation of a visual cue, which directed children’s attention towards their right or left hand, thus developing the anticipation of tactile stimulation. A regionally-specific prestimulus desynchronization of the alpha-range mu rhythm was observed over central electrode sites (C3/C4) contralateral to the cued direction. The authors suggested that anticipatory mu desynchronization might be regarded as a specific neural marker of attention focusing in young children. Our previous study (Talalay et al., 2018) was dedicated to the analysis of alpha-band functional connectivity during cued vs. implicit anticipation of visual and auditory stimuli. For cued anticipatory attention, an increase of functional links was observed between the intraparietal and prefrontal cortical areas (as compared with both the baseline and implicit learning sessions), and it was more prominent in the right hemisphere. It was discovered that cued anticipatory attention was underlain by functional modality-specific cortical links in accordance with the modality of anticipated stimuli.
Despite a large number of behavioral studies on the development of spatial cued attention, there is little information on the brain’s functional organization during selective anticipatory attention in children. The functional organization of the cortex is usually studied by means of functional connectivity analysis – i.e., the analysis of “statistical dependencies among remote neurophysiological events” (Friston, 2011, p. 14).
In this regard, the goal of the present study was to explore modality-specific cued attention in 9- to 10-year-old children in terms of both prestimulus functional connectivity and poststimulus task performance.
METHODS
The present study is based on our previous research and shares common or analogous methods and types of analysis with (Talalay, Machinskaya, 2014) and (Talalay et al., 2018).
2.1. Participants
A total of 17 right-handed, healthy children (11 male, 6 female) aged 9.789 ± 0.447 years participated in the study. They had normal or corrected-to-normal vision, and reported no history of neurological disorders. All participants’ parents gave written informed consent after the task was explained. All experimental methods had ethical approval from the Ethics Committee of the Institute of Developmental Physiology.
2.2. Stimuli
Visual stimuli were light gray and dark gray elongated hexagons with 2.5° × 2.5° angular size presented one at a time with a stimulus onset asynchrony (SOA) of 90 ms at the center of a black display screen; the angle between hexagons was 90° (see Fig. 1 (a)). Each stimulus within the pair was presented for 15 ms.
Fig. 1.
Experimental material and procedures. (a) All four variants of the visual stimulus pairs used in the order judgment task. In each pair, the first stimulus is obscured by the second one. (b) Symbolic images of an ear and an eye are used to cue the modality of the upcoming stimulus pair. (c) Sequence of events in a typical trial of the cued attention session. (d) Sequence of events in a typical trial of the baseline session. Timing of all events is shown along the horizontal time axis.
Рис. 1. Стимульный материал и процедура экспериментального исследования: (a) – все варианты зрительных целевых пар стимулов (первый стимул в паре показан на заднем плане); (b) – схематические изображения глаза и уха, которые использовались для формирования у испытуемых предвосхищения сенсорной модальности целевых сигналов; (c) – последовательность событий в одной пробе сессии “Сued attention” (произвольное предвосхищение); (d) – последовательность событий в одной пробе сессии “Baseline” (референтное условие). Временные интервалы между событиями обозначены на горизонтальной оси t.
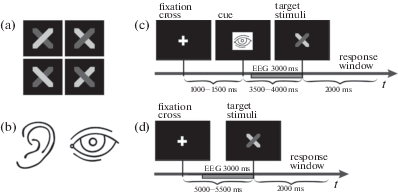
Participants performed the temporal order judgment task. The task was to decide which stimulus from the pair occurred first and then respond manually by pressing one of three buttons corresponding to the three possible alternatives: (a) a light gray hexagon occurred first, (b) a dark gray hexagon occurred first, or (c) I cannot give an answer. Participants had to respond within a 2-s response window. Auditory stimuli were short sounds of two different frequencies (300 and 3000 Hz) presented binaurally, one by one, at 50-ms intervals. Every stimulus from the pair was presented for 25 ms. The task was the same as for the visual stimuli. The interstimulus intervals and the duration of the target stimuli were defined after a preliminary experiment involving 15 children (9–10 years old). Participants performed both the visual and auditory tasks with an accuracy of 60%–70% correct when the described SOAs were applied.
2.3. Procedure
There were two successive experimental sessions that represented the cued attention and baseline conditions. The order of the two sessions was counterbalanced across participants. Furthermore, there was a short training session, which preceded the whole experiment.
In the cued attention session, the presentation of the target stimuli was preceded by a cue with a schematic image of an ear or an eye, which informed participants about the upcoming stimulus modality (see Fig. 1 (b)). Every experimental trial began with the presentation of a fixation cross at the center of the display. After that, one of the modality-specific cues was presented at 1000–1500 ms for 80 ms at the center of the computer display. Visual or auditory targets appeared at 3500–4000 ms after cueing. The sequence of auditory and visual stimuli was pseudorandom; a participant had 2 s to give a response. All participants responded with the index, middle or ring finger of the right (preferred) hand. The number of visual and auditory stimuli was equal (40 presentations); the session consisted of 80 trials. The order of events in one experimental trial is illustrated in Fig. 1 (c). The same target stimuli were used in both sessions.
The baseline session was designed so that participants could not anticipate the modality of an upcoming trial. The sequence of auditory and visual stimuli in this session was also pseudorandom. The number of visual and auditory stimuli was equal (40 presentations); the session included 80 trials. Unlike the cued attention session, the baseline session implied no cueing. The order of events in one experimental trial is illustrated in Fig. 1 (d).
2.4. Equipment and recording
The experiment was conducted in a darkened, soundproof room. Participants were seated in a comfortable chair 1 m in front of a computer monitor. Every stimulus was presented on a black display screen with a 60 Hz refresh rate. Two computers were connected over the TCP/IP protocol. One computer was used to manage the experimental procedure; the other one was a part of a 128-channel EEG system–EGI 300 (Electrical Geodesics, Inc., USA). The experiment was conducted with the help of custom software. This software allowed for the design of an experimental model and the control of an experimental process. Stimuli were presented via a computer display with a resolution of 800 × 600 pixels. Participants used a game pad (Genius.Usb-07) as a response device. The EEG was recorded from 128 electrodes (HydroCel GSN) referenced to the vertex at a sampling rate of 250 Hz with a passband between 0.5 and 70 Hz. All impedances were kept below 50 kΩ due to the specifications of the EGI’s amplifier (input impedance ≥ 200 MΩ).
2.5. Primary EEG analysis
EEG traces were recorded during 3 s before the presentation of the first target stimulus from the pair. We analyzed only those prestimulus EEG segments that corresponded to correct-response trials with excluded artifacts and power line (50 Hz) noise. Artifact EEG segments were detected visually and marked using graphical user interface software designed in GNU Octave. Artifact-free EEG segments were converted to the signals of separate cortical volume elements (voxels). For this, the EEG reference had been converted to the average of all channels. The signals from voxels were computed using minimum norm estimates. We used the functionality of the SPM8 software (Litvak et al., 2011). It is worth noting that we did not know either the skull and brain shape or exact electrode positions for each participant. That is why we used the ICBM-152 brain template from SPM8 and the average electrode positions for the 128-channel HydroCel GSN (www.egi.com). The inverse problem was solved in low resolution (cortical tissue was divided in 5124 voxels). Thus, the initial 128-channel recording (sensor space) was transformed into a 5124-channel recording (source space). From all 5124 voxel signals, we selected only those signals that corresponded to the regions of interest (ROI). ROI centers were defined with the help of SPM Anatomy toolbox v1.8 (https://www.ncbi.nlm.nih.gov/pubmed/15850749). The set of ROIs (see Table 1) was formed in accordance with our previous study (Talalay et al., 2018). The abbreviations of all ROIs presented in Table 1 are used throughout the rest of the text. We applied EEG-source alpha-band (7.5–12.5 Hz) coherence analysis to the pairs of the selected ROIs. The choice of this frequency band was underlain by the results of different EEG studies (Bastiaansen et al., 2001; Doesburg et al., 2009; Machinskaya et al., 1992; Rohenkohl, Nobre, 2011) indicating an important role of alpha-band oscillations in the neural mechanisms of anticipatory attention. All voxels that were located within a 10-mm radius sphere centered at a ROI center were regarded as referring to this ROI (see Table 1). We supposed that the total activity of these voxels (usually from 5 to 12 voxels) reflected the ROI-related processes. For the assessment of functional connectivity, each ROI was represented by its single characteristic signal. It was the first (with the highest eigenvalue) principal component computed using principal component analysis (PCA). All these characteristic signals formed a multichannel recording (the number of channels was equal to the number of ROIs) in EEG-source space.
Table 1.
MNI coordinates of the regions of interest Таблица 1. MNI-координаты областей интереса
ROI | Left hemisphere | Right hemisphere | ||||
---|---|---|---|---|---|---|
x | y | z | x | y | z | |
V1 – primary visual cortex (Brodmann area (BA) 17) | –9.8 | –88.2 | –8.1 | 9.8 | –88.2 | –8.1 |
A1 – primary auditory cortex (BA 41/42) | –40 | –32.8 | 14.6 | 40 | –32.8 | 14.6 |
pSMA – presupplementary motor area (BA 6a) | –6 | 6 | 70 | 9 | 6 | 70 |
VPC – ventral premotor cortex (ventral part of BA 6a) | –28 | –2 | 50 | 32 | –2 | 50 |
IPC – intraparietal cortex (part of BA 40) | –51.6 | –55.9 | 44.9 | 51.6 | –55.9 | 44.9 |
LPFC – lateral prefrontal cortex (BA 9/46) | –44 | 40 | 20 | 44 | 40 | 20 |
FEF – frontal eye field (BA 8) | –30 | –4 | 60 | 30 | –4 | 60 |
2.6. EEG-source coherence analysis
In order to avoid biased estimates, we used the following procedure (Kurgansky, 2010). All 14-channel recordings (according to the number of ROIs) that consisted of different-sized segments (see Section 2.6) were subdivided into adjacent (and nonoverlapping) 200-ms intervals. Thus, we derived a certain number of 200-ms segments that was different for each participant and experimental condition. This difference depended on the number and length of artifact segments. We chose a standard total length of artifact-free EEG for each participant that equaled 2 s (ten 200-ms segments). For each 2-s epoch, we estimated the coefficients of a vector autoregression (VAR) model of order 14 using the method described in (Cui et al., 2008). The estimated VAR coefficients were used to measure coherence (COH) functions (Kurgansky, 2010). For each participant in each experimental condition, we obtained as many coherence values for as many 2-s epochs that were formed. These values were averaged so that each participant in each experimental condition was characterized by a single set of coherence functions. Further statistical testing was performed on alpha-band (7.5–12.5 Hz) coherence values using the general linear model (GLM). The multivariate criteria of statistical significance (Wilks’s lambda) were used. Individual alpha-band limits were determined using the procedure described in (Machinskaya, Kurgansky, 2012).
RESULTS
3.1. Task performance
Unfortunately, two participants failed to respond in a designated time during the baseline and cued attention sessions, which is why it was impossible to identify their RT values and accuracy scores. The data obtained from those subjects were excluded from further analysis. Thus, the data for 15 participants were studied.
To analyze the influence of valid cueing on task performance, we tested RT values and accuracy scores (the percentage of correct responses) using a GLM with modality (visual, auditory) and experimental condition (baseline condition, cued anticipation) as the within-subject factors.
3.1.1. Accuracy
The statistical analysis showed no main effects and no significant interaction of the factors. In Fig. 2 (b), accuracy is shown separately for each cross-condition.
Fig. 2.
(a) Averaged RT in milliseconds and (b) accuracy (percentage of correct responses) for visual and auditory modalities are shown for two experimental conditions. Error bars correspond to the standard error of mean (SEM).
Рис. 2. Среднее время реакции (ВР) в миллисекундах (a) и усредненные проценты правильных ответов (b) представлены для каждого условия и сенсорной модальности. Планки погрешности соответствуют величине стандартной ошибки среднего (SEM).
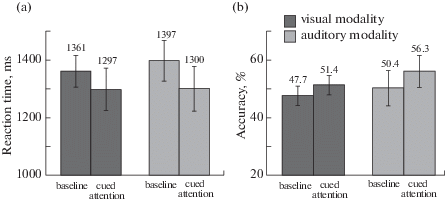
3.1.2. Reaction time
The analysis of variance revealed a main effect of condition, F(1, 14) = 4.660, p = 0.049, $\eta _{p}^{2}$ = = 0.250 and no significant interaction of condition and modality. In Fig. 2 (a), RT is shown separately for each cross-condition. Mean RT values and mean accuracy scores for each cross-condition are shown in Table 2.
Table 2.
Mean RT values and accuracy scores with standard deviations for each cross-condition Таблица 2. Среднее ВР и усредненные проценты правильных ответов со стандартными отклонениями представлены для каждого условия и сенсорной модальности
Condition | Visual modality | Auditory modality | ||
---|---|---|---|---|
RT (ms) | Accuracy (percentage of correct responses) | RT (ms) | Accuracy (percentage of correct responses) | |
Baseline | 1361 ± 214 | 47.7 ± 13 | 1397 ± 274 | 50.4 ± 23.7 |
Cued attention | 1297 ± 283 | 51.4 ± 12.9 | 1300 ± 301 | 56.3 ± 21.5 |
3.2. Functional connectivity
The data belonging to one participant were of poor quality and were excluded from the analysis of functional connectivity. The data for two other subjects had been excluded during the analysis of task performance. Thus, the data for 14 participants were analyzed.
Considering that we studied only interhemispheric functional links between the cortices, there were 21 pairs of ROIs in each hemisphere. A total of 42 pairs were grouped in five subsets for further analysis in accordance with our previous study (Talalay et al., 2018), because the size of the sample was insufficient to test all 42 pairs using the same GLM procedure. The five subsets are described below:
• Subset 1 represents functional links between the primary visual, primary auditory, parietal, and central cortices: V1–IPC, V1–A1, V1–pSMA, V1–VPC;
• Subset 2 represents functional links between the primary auditory, parietal, frontal, and central cortices: A1–pSMA, A1–VPC, A1–LPFC, A1–FEF, A1–IPC;
• Subset 3 represents functional links between the primary visual, parietal, and prefrontal cortices: V1–LPFC, V1–FEF, IPC–LPFC, IPC–FEF;
• Subset 4 represents centroparietal functional links: IPC–pSMA, IPC–VPC, pSMA–VPC;
• Subset 5 represents frontocentral functional links: pSMA–LPFC, pSMA– FEF, VPC–LPFC, VPC–FEF, LPFC–FEF.
For each subset, we used a GLM with experimental condition (cued attention, baseline), hemisphere (left, right), modality (visual, auditory), and localization (all pairs of ROIs included in a subset) as the within-subject factors. In case of a significant interaction of condition and localization alone or in combination with modality and hemisphere, the effect of condition for each pair of ROI was analyzed with a probability criterion p < 0.0167 (0.05/3; for Subset 4), p < 0.0125 (0.05/4; for Subsets 1, 3) or p < 0.0100 (0.05/5; for Subset 2, 5) depending on the number of ROI pairs in each subset to provide multiple testing correction.
The results of the GLM analysis for each subset are presented in Table 3 and described in detail below. Coherence values for each cross-condition are shown in Fig. 3 separately for each ROI pair in each subset.
Table 3.
GLM results for alpha-band coherence values. Significant and nearly significant effects and interactions Таблица 3. Результаты GLM-анализа когерентности альфа-ритма (альфа-КОГ). Значимые (и значимые на уровне тенденции) эффекты и взаимодействия
Factors | Subset 1 | Subset 2 | Subset 3 | Subset 4 | Subset 5 |
---|---|---|---|---|---|
Condition | F(1, 13) = 4.241, p = 0.060, $\eta _{p}^{2}$ = 0.246 |
– | – | F(1, 13) = 6.467, p = 0.025, $\eta _{p}^{2}$ = 0.332 | – |
Condition × Hemisphere | F(1, 13) = 4.154, p = 0.062, $\eta _{p}^{2}$ = 0.242 |
– | – | – | – |
Condition × Modality | – | – | – | – | – |
Condition × Localization | F(3, 11) = 8.751, p = 0.003, $\eta _{p}^{2}$ = 0.705 | – | – | – | – |
Condition × Localization × Modality | – | – | – | – | – |
Condition × Hemisphere × Modality | F(1, 13) = 3.457, p = 0.086, $\eta _{p}^{2}$ = 0.210 | – | F(1, 13) = 6.434, p = 0.025, $\eta _{p}^{2}$ = 0.331 | – | – |
Condition × Hemisphere × × Localization | – | F(4, 10) = 2.702, p = 0.092, $\eta _{p}^{2}$ = 0.519 | – | – | – |
Condition × Hemisphere × Modality × × Localization | – | – | – | – | – |
Fig. 3.
Average COH values are shown for each subset and each cross-condition. Error bars represent the standard error of mean (SEM). Significant differences (ps < 0.0167, 0.0125, or 0.0100) are marked with stars.
Рис. 3. Средние значения альфа-КОГ в парах областей интереса представлены для каждого подмножества, полушария и сенсорной модальности. Планки погрешности соответствуют величине стандартной ошибки среднего (SEM). Значимые различия (при p < 0.0167, 0.0125 или 0.0100) обозначены звездочками (*).
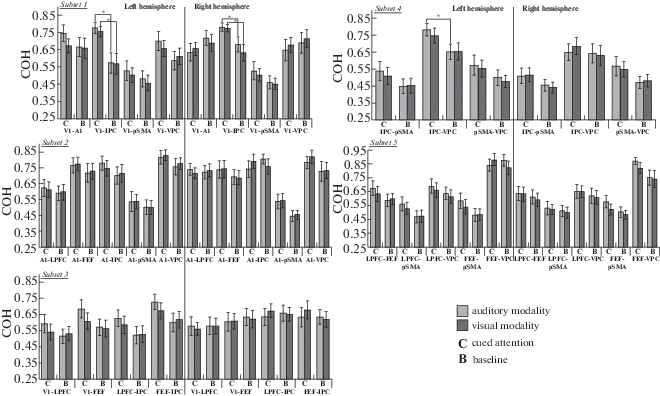
3.2.1. Subset 1: links between the primary visual, primary auditory, parietal, and central cortices
The analysis of variance revealed a nearly significant effect of condition (Table 3). It was shown that COH values during cued attention (M = 0.659, SD = 0.160) were higher than those during the baseline condition (M = 0.602, SD = = 0.186). Furthermore, the analysis showed a significant interaction of condition and localization, a nearly significant interaction of condition and hemisphere, and a nearly significant interaction of hemisphere, condition and modality (Table 3). Then we performed statistical tests with condition, modality, and localization as the within-subject factors separately for the left and right hemispheres.
Left hemisphere
The GLM analysis showed a significant effect of condition, F(1, 13) = 7.732, p = 0.016, $\eta _{p}^{2}$ = 0.373. COH values during cued attention (M = 0.667, SD = 0.165) were higher than those during the baseline condition (M = 0.575, SD = 0.199). Furthermore, the GLM analysis showed a significant interaction of condition and localization, F(3, 11) = 4.795, p = 0.023, $\eta _{p}^{2}$ = 0.567. Then we tested the condition factor separately for each ROI pair in both modalities.
For V1–IPC (Fig. 3), a significant effect of condition was found both in the auditory, F(1, 13) = = 21.306, p < 0.0001, $\eta _{p}^{2}$ = 0.621, and in the visual modalities, F(1, 13) = 16.641, p = 0.001, $\eta _{p}^{2}$ = = 0.561. For the auditory modality, COH values during cued attention (M = 0.776, SD = 0.129) were higher than those during the baseline condition (M = 0.572, SD = 0.219). For the visual modality, COH values during cued attention (M = = 0.754, SD = 0.115) were also higher than COH values during the baseline condition (M = 0.568, SD = 0.227).
Right hemisphere
The GLM analysis showed a significant interaction of condition and localization, F(3, 11) = = 6.131, p = 0.010, $\eta _{p}^{2}$ = 0.626. Then we tested the condition factor separately for each ROI pair in both modalities.
For V1–IPC (Fig. 3), a significant effect of condition was found both in the auditory, F(1, 13) = = 8.427, p = 0.012, $\eta _{p}^{2}$ = 0.393, and in the visual modalities, F(1, 13) = 10.976, p = 0.006, $\eta _{p}^{2}$ = = 0.458. For the auditory modality, COH values during cued attention (M = 0.781, SD = 0.091) were higher than those during the baseline condition (M = 0.680, SD = 0.161). For the visual modality, COH values during cued attention (M = = 0.775, SD = 0.079) were also higher than COH values during the baseline condition (M = 0.632, SD = 0.182).
3.2.2. Subset 2: links between the primary auditory, parietal, frontal, and central cortices
For this subset, a nearly significant interaction of condition, localization and hemisphere was found (Table 3). Because of that, we performed the GLM analysis separately for each hemisphere. The analysis showed no effect of condition and no significant interactions of condition and the other within-subject factors in both hemispheres.
3.2.3. Subset 3: links between the primary visual, parietal, and prefrontal cortices
The analysis of variance revealed a significant interaction of condition, hemisphere and modality (Table 3). Then we performed statistical tests with condition, modality, and localization as the within-subject factors separately for the left and right hemispheres. In the right hemisphere, no significant effects and interactions were found. In the left hemisphere, a nearly significant effect of condition was observed, F(1, 13) = 4.615, p = 0.051, $\eta _{p}^{2}$ = 0.262. COH values during cued attention (M = 0.631, SD = 0.194) were higher than those during the baseline condition (M = 0.557, SD = = 0.189). For the cued attention condition, we used a GLM with hemisphere, modality, and localization as the within-subject factors. The statistical analysis showed a significant interaction of modality and hemisphere, F(1, 13) = 5.055, p = = 0.043, $\eta _{p}^{2}$ = 0.280. For each sensory modality, the analysis of variance with hemisphere and localization as the within-subject factors showed no effect of hemisphere and no interaction of hemisphere and localization during cued attention. According to Fig. 3, the effect of condition in the left hemisphere might be more pronounced during auditory cued attention. To check this assumption, we used a GLM with condition and localization as the within-subject factors separately for each sensory modality. For the visual modality, no main effect of condition and no significant interaction of condition and localization were found. For the auditory modality, the statistical analysis showed a significant effect of condition, F(1, 13) = 9.283, p = 0.009, $\eta _{p}^{2}$ = 0.417. COH values during auditory cued attention (M = 0.659, SD = 0.198) were higher than those during the baseline condition (M = 0.553, SD = 0.194). Although no significant interaction of condition and localization was found, we performed post hoc analyses in order to determine the magnitude of the condition effect at the level of separate ROI pairs. The correction of multiple testing (nonsignificant: p ≥ 0.05/4) was applied to reduce the probability of false positive results. For each pair of ROIs in the left hemisphere, no significant effect of condition was observed for the auditory modality.
3.2.4. Subset 4: centroparietal links
For this subset, the statistical analysis revealed a main effect of condition (Table 3). COH values during cued attention (M = 0.600, SD = 0.182) were higher than those during the baseline condition (M = 0.527, SD = 0.162). Despite the absence of any interactions of condition and the other within-subject factors, we performed post hoc analyses in order to determine the magnitude of the condition effect at the level of separate ROI pairs. The correction of multiple testing (nonsignificant: p ≥ 0.05/3) was applied to reduce the probability of false positive results. Thus, we performed the GLM analysis separately for the left and right hemispheres. In the right hemisphere, no significant effects and interactions were observed. In the left hemisphere, a main effect of condition was found, F(1, 13) = 5.729, p = 0.032, $\eta _{p}^{2}$ = 0.306. As expected, COH values during cued attention (M = 0.619, SD = 0.186) were higher than those during the baseline condition (M = = 0.532, SD = 0.167). Then we tested the condition factor in both modalities separately for each ROI pair in the left hemisphere. For IPC–VPC (Fig. 3), a significant effect of condition, F(1, 13) = = 10.480, p = 0.006, $\eta _{p}^{2}$ = 0.446, was observed in the auditory modality. COH values during cued attention (M = 0.784, SD = 0.133) were higher than those during the baseline condition (M = = 0.654, SD = 0.169).
3.2.5. Subset 5: frontocentral links
The GLM analysis (Table 3) showed no effect of condition and no significant interactions of condition and the other within-subject factors.
DISCUSSION
The behavioral data analysis showed a positive influence of valid cueing on task performance: a significant decrease of RT was observed in the cued attention condition. This finding is concordant with the results of numerous studies (Mezzacappa, 2004; Rueda et al., 2004; Santhana Gopalan et al., 2019; Swanson et al., 1991). Fig. 2 (a) shows that the improvement of RT values was more pronounced for the auditory modality. This might be linked to the difference in the selection of visual and auditory information described in (Neumann et al., 1986). According to O. Neumann and colleagues, an auditory selection system has limited capacity and is restricted to one auditory event at a time. Auditory events are usually isolated, simple and clear-cut patterns, which include interwoven pieces of relevant information and irrelevant noise. At the same time, a visual selection system usually deals with multiple spatially separated stimuli, which are continuously available from all visible surfaces. In vision, the main task of the selection mechanisms is to decide which of the many alternative objects of attention should be selected (the problem of choice). In audition, the main task of the selection mechanisms is to decide whether or not an event in the environment should be selected (the problem of signal-noise separation). Thus, auditory attention might be more suited (in comparison with visual attention) to select signals from the noise and to detect the order of successively presented stimuli.
In comparison with the present study, our previous research on anticipatory attention in adults (Talalay, Machinskaya, 2014; Talalay et al., 2018) showed no significant impact of cueing on either performance accuracy or response rate. It seems that adults rely on external conditioning less than children do during the deployment of cued attention. Indeed, the adults showed better task performance in both the baseline and cued attention conditions; however, the children’s cue-related improvement in task performance was more pronounced. Nevertheless, this assumption requires additional research to be done.
Concerning alpha-band functional connectivity, the results of the present study indicate the strengthening of functional links between the primary visual and intraparietal cortices. These functional links were observed in both hemispheres during the anticipation of visual and auditory stimuli (Fig. 4). Furthermore, an increase of functional interaction between the intraparietal cortex and the ventral premotor cortex was observed only in the left hemisphere during auditory anticipatory attention.
Fig. 4.
A significant increase of COH values in the prestimulus period during cued attention, as compared with the baseline condition. Black lines show functional links between the cortical areas.
Рис. 4. Значимое увеличение альфа-КОГ в предстимульный период при произвольном предвосхищении по сравнению с референтным условием. Черные линии отражают функциональные связи между областями интереса.
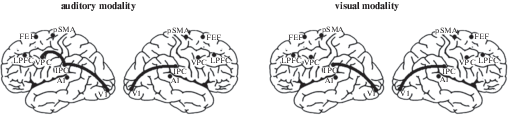
In comparison with adults (Talalay et al., 2018), the analysis of functional connectivity during cued attention in children showed no involvement of the frontoparietal network in the right hemisphere. The analysis of variance showed even no effect of condition and no interaction of condition and the other within-subject factors in the right hemisphere for the subset consisting of distant frontocaudal ROI pairs: V1–LPFC, V1–FEF, IPC–LPFC, and IPC–FEF. This finding is concordant with the results of various studies suggesting that the development of exogenous cued attention is related to the maturation (Santhana Gopalan et al., 2019) of the frontal and parietal areas (Capotosto et al., 2009, 2012; Liu et al., 2016; Shomstein, 2012; Simpson et al., 2011) of the right hemisphere (Corbetta, Shulman, 2002; Talalay et al., 2018). According to T. Zanto and colleagues (Zanto et al., 2011), the dorsolateral prefrontal cortex of the right hemisphere mediates top-down control of anticipatory processes and subsequent retention of information in working memory. The authors found that the activation of the inferior frontal junction of the right hemisphere was linked to the strengthening of alpha-band (7–14 Hz) functional links between the prefrontal and parietal cortices. Furthermore, P. Santhana Gopalan and colleagues (2019) suggested that the maintenance of alertness and readiness matures only in late childhood (after 12 years of age). According to the authors, frontoparietal activity in adults might reflect more top-down control of attention that is not utilized by children (Casey et al., 2004). A developmental EEG study on intrahemispheric functional connectivity during tactile and auditory anticipatory attention (Dubrovinskaya et al., 2000) corroborates the idea that the “regulatory” specificity of the right hemisphere is still immature in children aged 9 years. As mentioned above, the present study revealed that the strengthening of functional interaction between the intraparietal cortex and the ventral premotor cortex was only in the left hemisphere. This finding as well as the absence of frontoparietal interactions in the right hemisphere is concordant with an EEG (Thatcher, 1994) and an fMRI (Sherman et al., 2014) study indicating prolonged (up to adolescence) development of frontoparietal resting-state functional links in the right hemisphere.
In our previous study (Talalay et al., 2018), the analysis of functional connectivity in adults revealed modality-specific interactions of cortical areas during cued attention. The anticipation of auditory stimuli was reflected in functional links between the right LPFC and primary auditory cortex; the anticipation of visual stimuli was accompanied by the strengthening of functional links between the primary visual cortex and either the right LPFC or the left FEF. At the same time, no modality-specific functional links were found in the current study. The results support the notion that the deployment of modality-specific anticipatory attention in adults is determined by top-down modulatory influences of the frontal and parietal cortices on sensory cortical activity (Bressler et al., 2008; Simpson et al., 2011).
CONCLUSION
The present study revealed alpha-band functional connectivity during modality-specific anticipatory attention in children aged 9–10 years. As compared with the baseline condition, an increase of local functional links between the primary visual and intraparietal cortices was observed in both hemispheres during the anticipation of visual and auditory stimuli. The strengthening of functional interaction between the intraparietal cortex and the ventral premotor cortex was observed only in the left hemisphere during auditory anticipatory attention. Unlike our previous research on anticipatory attention in adults, the analysis of functional connectivity in children showed no frontoparietal functional links in the right hemisphere and no modality-specific cortical links. The results of the present study suggest that the brain’s top-down modulatory systems of the right hemisphere are still immature in children aged 9–10 years.
Список литературы
Akhtar N., Enns J.T. Relations between convert orienting and filtering in the development of visual attention. Journal of Experimental Child Psychology. 1989. 48 (2): 315–334. https://doi.org/10.1016/0022-0965(89)90008-8
Altamura M., Carver F.W., Elvevåg B., Weinberger D.R., Coppola R. Dynamic cortical involvement in implicit anticipation during statistical learning. Neuroscience Letters. 2014. 558: 73–77. https://doi.org/10.1016/j.neulet.2013.09.043
Bares M., Rektor I. Basal ganglia involvement in sensory and cognitive processing. A depth electrode CNV study in human subjects. Clinical Neurophysiology. 2001. 112 (11): 2022–2030. https://doi.org/10.1016/S1388-2457(01)00671-X
Bastiaansen M.C.M., Böcker K.B.E., Brunia C.H.M., De Munck J.C., Spekreijse H. Event-related desynchronization during anticipatory attention for an upcoming stimulus: A comparative EEG/MEG study. Clinical Neurophysiology. 2001. 112 (2): 393–403.https://doi.org/10.1016/S1388-2457(00)00537-X
Bastiaansen M.C.M., Brunia C.H.M. Anticipatory attention: An event-related desynchronization approach. International Journal of Psychophysiology. 2001. 43 (1): 91–107. https://doi.org/10.1016/S0167-8760(01)00181-7
Bressler S.L., Tang W., Sylvester C.M., Shulman G.L., Corbetta M. Top-down control of human visual cortex by frontal and parietal cortex in anticipatory visual spatial attention. Journal of Neuroscience. 2008. 28 (40): 10056–10061. https://doi.org/10.1523/JNEUROSCI.1776-08.2008
Bianco V., Berchicci M., Livio Perri R., Quinzi F., Mussini E., Spinelli D., Di Russo F. Preparatory ERPs in visual, auditory, and somatosensory discriminative motor tasks. Psychophysiology, 2020. 57 (12): e13687. https://doi.org/10.1111/psyp.13687
Brunia C.H.M., Damen E.J.P. Distribution of slow brain potentials related to motor preparation and stimulus anticipation in a time estimation task. Electroencephalography and Clinical Neurophysiology. 1988. 69 (3): 234–243. https://doi.org/10.1016/0013-4694(88)90132-0
Brunia C.H.M., Hackley S.A., van Boxtel G.J.M., Kotani Y., Ohgami Y. Waiting to perceive: Reward or punishment? Clinical Neurophysiology. 2011. 122 (5): 858–868. https://doi.org/10.1016/j.clinph.2010.12.039
Brunia C.H.M., van Boxtel G.J.M. Anticipatory attention to verbal and non-verbal stimuli is reflected in a modality-specific SPN. Experimental Brain Research. 2004. 156 (2): 231–239. https://doi.org/10.1007/s00221-003-1780-2
Capotosto P., Babiloni C., Romani G.L., Corbetta M. Differential contribution of right and left parietal cortex to the control of spatial attention: A simultaneous EEG-rTMS study. Cerebral Cortex. 2012. 22 (2): 446–454. https://doi.org/10.1093/cercor/bhr127
Capotosto P., Babiloni C., Romani G.L., Corbetta M. Frontoparietal cortex controls spatial attention through modulation of anticipatory alpha rhythms. Journal of Neuroscience. 2009. 29 (18): 5863–5872. https://doi.org/10.1523/JNEUROSCI.0539-09.2009
Casey B.J., Davidson M.C., Hara Y., Thomas K.M., Martinez A., Galvan A., Halperin J.M., Rodríguez-Aranda C.E., Tottenham N. Early development of subcortical regions involved in non-cued attention switching. Developmental Science. 2004. 7 (5): 534–542. https://doi.org/10.1111/j.1467-7687.2004.00377.x
Cleeremans A., Destrebecqz A., Boyer M. Implicit learning: News from the front. Trends in Cognitive Sciences. 1998. 2 (10): 406–416. https://doi.org/10.1016/S1364-6613(98)01232-7
Corbetta M., Shulman G.L. Control of goal-directed and stimulus-driven attention in the brain. Nature Reviews Neuroscience. 2002. 3 (3): 201–215. https://doi.org/10.1038/nrn755
Cui J., Xu L., Bressler S.L., Ding M., Liang H. BSMART: A Matlab/C toolbox for analysis of multichannel neural time series. Neural Networks. 2008. 21 (8): 1094–1104. https://doi.org/10.1016/j.neunet.2008.05.007
Dale R., Duran N., Morehead R. Prediction during statistical learning, and implications for the implicit/explicit divide. Advances in Cognitive Psychology. 2012. 8 (2): 196–209. https://doi.org/10.5709/acp-0115-z
Damen E.J.P., Brunia C.H.M. Changes in Heart Rate and Slow Brain Potentials Related to Motor Preparation and Stimulus Anticipation in a Time Estimation Task. Psychophysiology. 1987. 24 (6): 700–713. https://doi.org/10.1111/j.1469-8986.1987.tb00353.x
Di Russo F., Berchicci M., Bozzacchi C., Perri R.L., Pitzalis S., Spinelli D. Beyond the “Bereitschaftspotential”: Action preparation behind cognitive functions. Neuroscience and Biobehavioral Reviews. 2017. 78: 57–81. https://doi.org/10.1016/j.neubiorev.2017.04.019
Doesburg S.M., Green J.J., McDonald J.J., Ward L.M. From local inhibition to long-range integration: A functional dissociation of alpha-band synchronization across cortical scales in visuospatial attention. Brain Research. 2009. 1303: 97–110. https://doi.org/10.1016/j.brainres.2009.09.069
Dubrovinskaya N.V., Machinskaya R.I., Kulakovsky Y.V. Brain Organization of Selective Tasks Preceding Attention: Ontogenetic Aspects. Complex Brain Functions. Conceptual Advances in Russian Neuroscience. Ed. Miller R., Ivanitsky A.M., Balaban P.M. Harwood Academic Publishers, 2000. 168–180 pp.
Enns J.T., Brodeur D.A. A developmental study of covert orienting to peripheral visual cues. Journal of Experimental Child Psychology. 1989. 48 (2): 171–189. https://doi.org/10.1016/0022-0965(89)90001-5
Eriksen B.A., Eriksen C.W. Effects of noise letters upon the identification of a target letter in a nonsearch task. Perception & Psychophysics. 1974. 16 (1): 143–149. https://doi.org/10.3758/BF03203267
Fan J., Kolster R., Ghajar J., Suh M., Knight R.T., Sarkar R., McCandliss B.D. Response anticipation and response conflict: An event-related potential and functional magnetic resonance imaging study. Journal of Neuroscience. 2007. 27 (9): 2272–2282. https://doi.org/10.1523/JNEUROSCI.3470-06.2007
Fan J., McCandliss B.D., Fossella J., Flombaum J.I., Posner M.I. The activation of attentional networks. NeuroImage. 2005. 26 (2): 471–479. https://doi.org/10.1016/j.neuroimage.2005.02.004
Fan J., McCandliss B.D., Sommer T., Raz A., Posner M.I. Testing the efficiency and independence of attentional networks. Journal of Cognitive Neuroscience. 2002. 14 (3): 340–347. https://doi.org/10.1162/089892902317361886
Folk C.L., Hoyer W.J. Aging and shifts of visual spatial attention. Psychology and Aging. 1992. 7 (3): 453–465. https://doi.org/10.1037/0882-7974.7.3.453
Friston K.J. Functional and Effective Connectivity: A Review. Brain Connectivity. 2011. 1 (1): 13–36. https://doi.org/10.1089/brain.2011.0008
Gómez C.M., Marco J., Grau C. Preparatory visuo-motor cortical network of the contingent negative variation estimated by current density. NeuroImage. 2003. 20 (1): 216–224. https://doi.org/10.1016/S1053-8119(03)00295-7
Guo Z., Tan X., Pan Y., Liu X., Zhao G., Wang L., Peng Z. Contingent negative variation during a modified cueing task in simulated driving. PLoS ONE. 2019. 14 (11): e0224966. https://doi.org/10.1371/journal.pone.0224966
Hahn E., Ta T.M.T., Hahn C., Kuehl L.K., Ruehl C., Neuhaus A.H., Dettling M. Test-retest reliability of Attention Network Test measures in schizophrenia. Schizophrenia Research. 2011. 133 (1–3): 218–222. https://doi.org/10.1016/j.schres.2011.09.026
Jennings J.M., Dagenbach D., Engle C.M., Funke L.J. Age-related changes and the attention network task: An examination of alerting, orienting, and executive function. Aging, Neuropsychology, and Cognition. 2007. 14 (4): 353–369. https://doi.org/10.1080/13825580600788837
Jonkman L.M. The development of preparation, conflict monitoring and inhibition from early childhood to young adulthood: a Go/Nogo ERP study. Brain Research. 2006. 1097 (1): 181–193. https://doi.org/10.1016/j.brainres.2006.04.064
Jonkman L.M., Lansbergen M., Stauder J.E.A. Developmental differences in behavioral and event-related brain responses associated with response preparation and inhibition in a go/nogo task. Psychophysiology. 2003. 40 (5): 752–761. https://doi.org/10.1111/1469-8986.00075
Klimesch W. Alpha-band oscillations, attention, and controlled access to stored information. Trends in Cognitive Sciences. 2012. 16 (12): 606–617. https://doi.org/10.1016/j.tics.2012.10.007
Konrad K., Neufang S., Thiel C.M., Specht K., Hanisch C., Fan J., Herpertz-Dahlmann B., Fink G.R. Development of attentional networks: An fMRI study with children and adults. NeuroImage. 2005. 28 (2): 429–439. https://doi.org/10.1016/j.neuroimage.2005.06.065
Kurgansky A.V. Nekotorye voprosy issledovaniya kortiko-kortikal’nyh funkcional’nyh svyazej s pomoshch’yu vektornoj avtoregressionnoj modeli mnogokanal’noj EEG [Some methodological issues of studying cortico-cortical functional connectivity with vector autoregressive model of multichannel EEG]. Zh. Vyssh. Nerv. Deiat. im. I.P. Pavlova. 2010. 60 (6): 740–759.
Langley L.K., Friesen C.K., Saville A.L., Ciernia A.T. Timing of reflexive visuospatial orienting in young, young-old, and old-old adults. Attention, Perception, and Psychophysics. 2011. 73 (5): 1546–1561. https://doi.org/10.3758/s13414-011-0108-8
Leuthold H., Jentzsch I. Neural correlates of advance movement preparation: A dipole source analysis approach. Cognitive Brain Research. 2001. 12 (2): 207–224. https://doi.org/10.1016/S0926-6410(01)00052-0
Litvak V., Mattout J., Kiebel S., Phillips C., Henson R., Kilner J., Barnes G., Oostenveld R., Daunizeau J., Flandin G., Penny W., Friston K. EEG and MEG data analysis in SPM8. Computational Intelligence and Neuroscience. 2011. 2011: 1–32. https://doi.org/10.1155/2011/852961
Liu Y., Bengson J., Huang H., Mangun G.R., Ding M. Top-down Modulation of Neural Activity in Anticipatory Visual Attention: Control Mechanisms Revealed by Simultaneous EEG-fMRI. Cerebral Cortex. 2016. 26 (2): 517–529. https://doi.org/10.1093/cercor/bhu204
Machinskaya R.I., Machinskii N.O., Deryugina E.I. Funktsional’naia organizatsiia pravogo i levogo polushariǐ mozga cheloveka pri napravlennom vnimanii. Fiziologiia Cheloveka. 1992. 18 (6): 77–85.
Machinskaya R.I. Selective prestimulus attention: Two kinds of processing – two types of brain cortex functional organization. Downward processes in the perception representation mechanisms. Proceedings of the International School of Biocybernetics. Ed. Taddei-Ferretti C., Musio C. Singapore: World Scientific, 1998. 471–475 pp.
Machinskaya R.I., Kurgansky A.V. A comparative electrophysiological study of regulatory components of working memory in adults and seven- to eight-year-old children: An analysis of coherence of EEG rhythms. Human Physiology. 2012. 38 (1): 1–13. https://doi.org/10.1134/S0362119712010136
Mezzacappa E. Alerting, orienting, and executive attention: Developmental properties and sociodemographic correlates in an epidemiological sample of young, urban children. Child Development. 2004. 75 (5): 1373–1386. https://doi.org/10.1111/j.1467-8624.2004.00746.x
Morrison F.J. The development of alertness. Journal of Experimental Child Psychology. 1982. 34 (2): 187–199. https://doi.org/10.1016/0022-0965(82)90041-8
Mozolic J.L., Joyner D., Hugenschmidt C.E., Peiffer A.M., Kraft R.A., Maldjian J.A., Laurienti P.J. Cross-modal deactivations during modality-specific selective attention. BMC Neurology. 2008. 8: 35. https://doi.org/10.1186/1471-2377-8-35
Näätänen R. Attention and brain function. Hillsdale, NJ: Lawrence Erlbaum Associates, 1992.
Neumann O., van der Heijden A.H.C., Allport D.A. Visual selective attention: Introductory remarks. Psychological Research. 1986. 48 (4): 185–188. https://doi.org/10.1007/BF00309082
Perchet C., García-Larrea L. Visuospatial attention and motor reaction in children: An electrophysiological study of the “Posner” paradigm. Psychophysiology. 2000. 37 (2): 231–241. https://doi.org/10.1017/S0048577200981368
Périn B., Godefroy O., Fall S., de Marco G. Alertness in young healthy subjects: An fMRI study of brain region interactivity enhanced by a warning signal. Brain and Cognition. 2010. 72 (2): 271–281. https://doi.org/10.1016/j.bandc.2009.09.010
Petersen S.E., Posner M.I. The attention system of the human brain: 20 years after. Annual Review of Neuroscience. 2012. 35: 73–89. https://doi.org/10.1146/-neuro-062111-150525
Posner M.I. Orienting of attention. The Quarterly Journal of Experimental Psychology. 1980. 32 (1): 3–25. https://doi.org/10.1080/00335558008248231
Posner M.I. Chronometric Explorations of Mind. Hillsdale, NJ: Lawrence Erlbaum Associates, 1978. 286 p.
Posner M.I., Fan J. Attention as an organ system. Topics in Integrative Neuroscience: From Cells to Cognition. Ed. Pomerantz J.R. Cambridge: Cambridge University Press, 2008. 31–61 pp. https://doi.org/10.1017/CBO9780511541681.005
Posner M.I., Petersen S.E. The attention system of the human brain. Annual Review of Neuroscience. 1990. 13: 25–42. https://doi.org/10.1146/annurev.ne.13.030190.000325
Posner M.I., Rothbart M.K., Rueda M.R. Developing attention and self-regulation in infancy and childhood. Neural Circuit Development and Function in the Brain. Ed. Rubenstein J.L.R., Pasko Rakic. Elsevier, 2013. 395–411 pp. https://doi.org/10.1016/B978-0-12-397267-5.00059-5
Rohenkohl G., Gould I.C., Pessoa J., Nobre A.C. Combining spatial and temporal expectations to improve visual perception. Journal of Vision. 2014. 14 (4): 8. https://doi.org/10.1167/14.4.8
Rohenkohl G., Nobre A.C. Alpha oscillations related to anticipatory attention follow temporal expectations. Journal of Neuroscience. 2011. 31 (40): 14076–14084. https://doi.org/10.1523/JNEUROSCI.3387-11.2011
Rosahl S.K., Knight R.T. Role of prefrontal cortex in generation of the contingent negative variation. Cerebral Cortex. 1995. 5 (2): 123–134. https://doi.org/10.1093/cercor/5.2.123
Rueda M.R., Fan J., McCandliss B.D., Halparin J.D., Gruber D.B., Lercari L.P., Posner M.I. Development of attentional networks in childhood. Neuropsychologia. 2004. 42 (8): 1029–1040. https://doi.org/10.1016/j.neuropsychologia.2003.12.012
Santhana Gopalan P.R., Loberg O., Hämäläinen J.A., Leppänen P.H.T. Attentional processes in typically developing children as revealed using brain event-related potentials and their source localization in Attention Network Test. Scientific Reports. 2019. 9 (1): 2940. https://doi.org/10.1038/s41598-018-36947-3
Schul R., Townsend J., Stiles J. The development of attentional orienting during the school-age years. Developmental Science. 2003. 6 (3): 262–272. https://doi.org/10.1111/1467-7687.00282
Segalowitz S.J., Davies P.L. Charting the maturation of the frontal lobe: An electrophysiological strategy. Brain and Cognition. 2004. 55 (1): 116–133. https://doi.org/10.1016/S0278-2626(03)00283-5
Sherman L.E., Rudie J.D., Pfeifer J.H., Masten C.L., McNealy K., Dapretto M. Development of the default mode and central executive networks across early adolescence: a longitudinal study. Developmental cognitive neuroscience. 2014. 10: 148–159. https://doi.org/10.1016/j.dcn.2014.08.002
Shibasaki H., Hallett M. What is the Bereitschaftspotential? Clinical Neurophysiology. 2006. 117 (11): 2341–2356. https://doi.org/10.1016/j.clinph.2006.04.025
Shomstein S. Cognitive functions of the posterior parietal cortex: Top-down and bottom-up attentional control. Frontiers in Integrative Neuroscience. 2012. 6: 38. https://doi.org/10.3389/fnint.2012.00038
Simpson G.V., Weber D.L., Dale C.L., Pantazis D., Bressler S.L., Leahy R.M., Luks T.L. Dynamic activation of frontal, parietal, and sensory regions underlying anticipatory visual spatial attention. Journal of Neuroscience. 2011. 31 (39): 13880–13889. https://doi.org/10.1523/JNEUROSCI.1519-10.2011
Spence C., Driver J. On measuring selective attention to an expected sensory modality. Perception and Psychophysics. 1997. 59 (3): 389–403. https://doi.org/10.3758/BF03211906
Swanson J.M., Posner M., Potkin S., Bonforte S., Youpa D., Fiore C., Cantwell D., Crinella F. Activating tasks for the study of visual-spatial attention in ADHD children: a cognitive anatomic approach. Journal of Child Neurology. 1991. 6 (1): 119–127. https://doi.org/10.1177/0883073891006001s12
Talalay I., Machinskaya R. The Comparative Study of Cued and Implicit Anticipatory Attention During the Performance of Visual and Auditory Versions of the Temporal Order Judgment Task. The Russian Journal of Cognitive Science. 2014. 1 (4): 58–65.
Talalay I.V., Kurgansky, A.V., Machinskaya, R.I. Alpha-band functional connectivity during cued versus implicit modality-specific anticipatory attention: EEG-source coherence analysis. Psychophysiology. 2018. 55 (12): e13269. https://doi.org/10.1111/psyp.13269
Tecce J.J. Contingent negative variation (CNV) and psychological processes in man. Psychological Bulletin. 1972. 77 (2): 73–108. https://doi.org/10.1037/h0032177
Thatcher R. Cyclic Cortical Reorganization: Origins of Human Cognitive Development. Human Behavior and Developing Brain. Ed. Dawson G., Fisher K. New York, London: The Guilford Press, 1994. 232–269 pp.
Turk-Browne N.B., Scholl B.J., Johnson M.K., Chun M.M. Implicit perceptual anticipation triggered by statistical learning. Journal of Neuroscience. 2010. 30 (33): 11177–11187. https://doi.org/10.1523/JNEUROSCI.0858-10.2010
Ulrich R., Leuthold H., Sommer W. Motor programming of response force and movement direction. Psychophysiology. 1998. 35 (6): 721–728. https://doi.org/10.1111/1469-8986.3560721
Wainwright A., Bryson S.E. The development of exogenous orienting: Mechanisms of control. Journal of Experimental Child Psychology. 2002. 82 (2): 141–155. https://doi.org/10.1016/S0022-0965(02)00002-4
Walter W.G., Cooper R., Aldridge V.J., McCallum W.C., Winter A.L. Contingent negative variation: An electric sign of sensori-motor association and expectancy in the human brain. Nature. 1964. 203 (4943): 380–384.https://doi.org/10.1038/203380a0
Weiss S.M., Meltzoff A.N., Marshall P.J. Neural measures of anticipatory bodily attention in children: Relations with executive function. Developmental Cognitive Neuroscience. 2018. 34: 148–158. https://doi.org/10.1016/j.dcn.2018.08.002
Williams R.S., Biel A.L., Wegier P., Lapp L.K., Dyson B.J., Spaniol J. Age differences in the Attention Network Test: Evidence from behavior and event-related potentials. Brain and Cognition. 2016. 102: 65–79. https://doi.org/10.1016/j.bandc.2015.12.007
Zanto T.P., Rubens M.T., Thangavel A., Gazzaley A. Causal role of the prefrontal cortex in top-down modulation of visual processing and working memory. Nature Neuroscience. 2011. 14 (5): 656–663. https://doi.org/10.1038/nn.2773
Zhao J., Al-Aidroos N., Turk-Browne N.B. Attention Is Spontaneously Biased Toward Regularities. Psychological Science. 2013. 24 (5): 667–677. https://doi.org/10.1177/0956797612460407
Zhou S.-S., Fan J., Lee T.M.C., Wang C.-Q., Wang K. Age-related differences in attentional networks of alerting and executive control in young, middle-aged, and older Chinese adults. Brain and Cognition. 2011. 75 (2): 205–210. https://doi.org/10.1016/j.bandc.2010.12.003
Дополнительные материалы отсутствуют.
Инструменты
Журнал высшей нервной деятельности им. И.П. Павлова