Журнал высшей нервной деятельности им. И.П. Павлова, 2021, T. 71, № 4, стр. 515-528
A Longitudinal Study of Electroencephalogram Spatial Connectivity Maturation in Children and Adolescents-Northerners (From 8 to 16/17 Years Old)
N. V. Shemyakina a, *, **, Zh. V. Nagornova a, S. I. Soroko a
a Sechenov Institute of Evolutionary Physiology and Biochemistry, Russian Academy of Sciences
St. Petersburg, Russia
* E-mail: shemyakina_n@mail.ru
** E-mail: natalia.shemyakina@iephb.ru
Поступила в редакцию 30.11.2020
После доработки 1.03.2021
Принята к публикации 2.03.2021
Аннотация
This study aimed to reveal and describe the typical and specific longitudinal dynamics of functional and effective connectivity by means of electroencephalogram (EEG) in normal children living in the European North of Russia, boys and girls. The eyes-closed resting state EEGs were recorded in 15 children at a yearly basis during the developmental period from 8 to 16–17 years. Age-related changes in EEG connectivity were explored by coherence (functional connectivity) and Granger causality (GC, considered as effective connectivity) analyses in frequency and time domains, which were carried out in delta (1.6–4 Hz), theta (4–7.5 Hz), alpha1 (7.5–9.5 Hz), alpha2 (9.5–12.5 Hz), beta1 (12.5–18 Hz), beta2 (18–30 Hz), and common (1.6–30 Hz) frequency bands. The coherence analysis revealed maturation effects reflected in an increased connectivity in all frequency bands. Most pronounced changes of EEG coherence were revealed in alpha2, beta1, and common frequency bands. The interhemispheric frontal-parietal functional connectivity increased both in boys and girls. Additionally, in boys, interhemispheric functional connectivity increased between the central and temporal areas in alpha2 and common bands. In girls, there was observed an increase in intrahemispheric anterior-posterior functional connectivity in the alpha2 frequency band. The changes in effective connectivity in boys indicate an increased bidirectional information flow (revealed by the GC analysis) from the default mode network (DMN) to the frontoparietal network (FPN) and vice versa. By contrast, in girls, the information flow increases from the frontal to parietal areas (FPN), and decreasing between the central and frontal areas (sensorimotor network). The data suggest different age-related trends in the maturation of connectivity between the brain networks and different role of top-down and bottom-up regulation processes in boys compared to girls.
Studies of brain electric activity showed their informativeness for the assessment of the normal and pathological human brain development (Farber et al., 1990, Segalowitz et al., 2010). Across childhood and adolescence, the brain undergoes massive morphological changes, such as cortical refinement, synaptic growth and pruning, myelination (Casey et al., 2000, Segalowitz, 2010, Kurth et al., 2013). Influenced both by genetic and environmental factors, these processes result in the maturation of neuronal networks, simple and complex brain functions. However, it is poorly understood how functional brain connectivity develops and what factors moderate it (Tsitseroshin et al., 2003, Chorlian et al., 2007). Neuronal oscillations are considered as the basic parameter that defines functioning and interacting between and within the modules of the brain networks and thereby the basic mechanism of development and cognitive processing (Buzsaki, 2006; Buzsaki, Draguhn, 2004; Singer, 1999).
In terms of networks, functional activity of the human brain could be described as organized into independently active but interconnected brain regions, networks. Among the best explored are the default mode network (DMN), frontoparietal network (FPN) and salience network (SN). The first of them strongly dominates during the rest state, the next two are engaged in the tasks’ demand (Raichle et al., 2001; Greicius, Menon, 2004; Briley et al., 2018). The dorsal and ventral attention networks, as well as the visual and limbic networks, are also under investigation now (Ryali et al., 2016).
Magnetic resonance imaging (fMRI) data supported the viewpoint that sensory systems (e.g., the visual system) mature by the end of the childhood, while the integrity of the high-order networks (e.g., the FPN and SN) develops throughout adolescence (Briley et al., 2018). A MEG study by the Schäfers (2014) group addressed the development of resting-state networks (including FPN and DMN) between the ages of 6 and 34 years and reported a linear age-related increase in the connectivity in alpha and beta bands within these networks (Schäfer et al., 2014). Quite a replicative effect of increasing connectivity was obtained in a study by Briley et al. (Briley et al., 2018). The authors suggested that the development of coordinated beta-band oscillations within and between the higher-order cognitive networks through adolescence might contribute to the developing abilities of adolescents to focus their attention and coordinate diverse aspects of mental activity (Briley et al., 2018).
EEG coherence is primarily a statistical measure of the consistency of phase differences between two signals from different locations, and therefore it allows the examination of linear coupling or synchronization between brain regions as a function of frequency. Coherence values range from 0 to 1, with 1 meaning a perfect agreement between phase differences and 0 meaning completely random phase differences (Chorlian et al., 2007). A high coherence between two electrodes is expected to indicate functional integration between neuronal populations (Nunez, Srinivasan, 2006).
If two cortical areas show very similar electric activities one can deduce that these two brain areas are somehow functionally interrelated as they do the same thing at the same time. The phase coherence is then a measure to quantify the similarity between two signals in the brain (Kurth et al., 2013).
A systematic description of EEG coherence patterns was provided by Thatcher et al. (1986) in a two-compartment model (TCM) of cortico-cortical associations, in which short and long neuronal fibers contribute differentially to EEG coherence as a function of the inter-electrode distance. It is suggested that coherence between proximate electrodes mainly reflects short white matter fibers (1–3 cm), whereas moderate to high coherence at long distances is supposed to reflect long-distance white matter fasciculi (up to 25 cm). The TCM appears to accommodate much of the existing coherence data (van Beijsterveldt et al., 1998, van Baal et al., 2001, Barry et al., 2005).
In addition to the assessment of functional connectivity, methods for estimating effective connectivity have recently been widely used (Astolfi et al., 2005). Granger causality (GC) is a statistical hypothesis that gives an opportunity to test the causal influence of one-time series to another (Wiener, 1956; Granger, 1969). In neuroscience, GC has been used to detect directed functional (i.e. causal) connectivity for various brain data (Malekpour et al., 2012; Dhamala et al., 2008; Zhou et al., 2009; Liao et al., 2010) to study the connectivity and information flow between different brain regions (Friston, 1994) or to find out how one brain area influences the other (see Pereda et al., 2005, Gourevitch et al., 2006, Bressler, Seth 2011). The spectral measures of causality could be used to explore the role of different rhythms in causal connectivity between brain regions during maturation.
In this study, we used two linear methods: coherence as the index of functional connectivity and Granger causality (GC) as the index of effective connectivity. Both coherence and GC can be informative for exploring developmental changes in children’s resting-state EEGs.
We hypothesized that different parameters of connectivity in a longitudinal study can provide additional and complementary information about the EEG genesis and developmental trends.
The aim of this study was to investigate longitudinal maturational changes and the effect of age and gender on EEG in normal children living in the north region of Russia. In this study the maturation of brain connectivity was explored via the EEG spectral coherence and Granger causality analyses. As the number of longitudinal studies is not that large, the data on spatial cortex electrical attitudes development can expand our understanding of the maturational processes in boys and girls.
METHODS
Subjects. A longitudinal EEG study was carried out during 8 years (2005–2013) with the participation of school children aged 8 to 16–17 years born from 1993 to 1998. Children and adolescents were recruited from a rural secondary school in the Northern region of Russia (60°58′ N, 40°14′ E) and took part in the study voluntarily. EEG examinations were conducted once or twice a year. The group for longitudinal data analysis included only those children (9 girls, 6 boys) who had missing data for no more than two years of during the entire period of observation. There were made over 146 EEG recordings from 15 right-handed (Oldfield, 1971) children taken into account for the longitudinal assessment (Soroko et al., 2014).
None of the participants showed neurological or psychiatric diseases and took any drugs affecting the CNS. All procedures were carried out in accordance with the Helsinki Declaration (1974) and its updates. The study was approved by the Ethics Committee at the Sechenov Institute of Evolutionary Physiology and Biochemistry of the Russian Academy of Sciences (IEPhB RAS).
Procedure. EEGs in the eyes-closed resting state were recorded for 3 minutes using a 21-channel EEG system Encephalan-131-03 (Medicom-MTD, Taganrog, Russia, http://www.medicom-mtd.com). We used sixteen silver chloride cup EEG electrodes that were positioned according to the 10–20 international system at sites Fp1, Fp2, F7, F3, F4, F8, T3, C3, C4, T4, T5, P3, P4, T6, O1, O2 referenced to the linked ears and fixed on the head surface by the cap. The ground electrode was placed on the right cheek. Electrode impedances were constantly kept below 10 kOhm, the input signals were digitized at a rate of 250 Hz, amplifier’s bandpass 0.5–70 Hz, notch filter 45–55 Hz; analyzed EEGs bandpass 1.6–30 Hz. Electrooculogram artifacts were extracted using the ICA procedure (as in Kozhushko et al., 2018). For the analysis, there were used 30 000 artifact-free time points (120 sec) in each record for both types of analysis, coherence and GC.
As connectivity measurements are influenced by the volume conductance, we used the average referenced montage that could reduce the common reference effect (Nunez et al., 1999, Gudmundsson et al., 2007, Hu et al., 2019). For GC estimation, we also transform data to the average reference. Coherence and causality measurements show differential resistance to the presence of signals from a common source affecting both channels: GC is adversely affected by common signals, while coherence increases in the presence of common signals (Cohen et al., 2018). Therefore, the causality estimation provides additional data not only on the direction of the information flow but most probably on direct connectivity between two channels (not affected by common signals).
EEG coherence data analysis. The data were analyzed using the WinEEG software (© V.A. Pono-marev, Ju.D. Kropotov). The register for the computer programs of RF № 2001610516, 08.05.2001). EEG coherence calculations were performed using the standard approach C2xy( f ) = = (Sxy( f ))2/(Sxx( f ) × Syy( f )) (Bendat, Piersol, 1986), where C2xy( f ) – normal coherence between x and y sites for the frequency ( f ), (Sxy( f ))2 – cross spectrum, Sxx( f ) and Syy( f ) – autospectra. We applied 2 s epochs, 50% overlap, and Hanning time-window. EEG coherence values were averaged in seven frequency bands: delta (1.6–4 Hz), theta (4–7 Hz), alpha1 (7–9.5 Hz), alpha2 (9.5–12.5 Hz), beta1 (12.5–18 Hz), beta2 (18–30 Hz), broadband/common (1.6–30 Hz).
The arrays of EEG coherence estimations averaged for each subject were then normalized using the transformations Y = log(X2/(1 – X2)) (Gevins, Remond 1987).
EEG Granger casual connectivity analysis. All estimations of GC were carried out in the Matlab using Multivariate Granger Causality (MVGC) Toolbox (Barnett, Seth, 2014) with the multivariate autoregressive (MVAR) model, and its order was 20. In 1969, Granger introduced the idea of G-causality as a formalization, in terms of linear regression modeling, of Wiener’s (and Akaike’s) intuition that X2 ‘causes’ X1 if knowing X2 helps predict the future of X1 (Granger, 1969; Seth, Edelman, 2007). According to G-causality, X2 causes X1 if the inclusion of past observations of X2 reduces the prediction error of X1 in a linear regression model of X1 and X2 as compared to a model that includes only previous observations of X1 (Seth, 2010). A frequency-domain (spectral) interpretation of G-causality was derived by examining the Fourier components of an MVAR model estimated in the time-domain (Geweke, 1982). A spectral G-causality indexes were estimated in delta (1.6–4 Hz), theta (4–7 Hz), alpha1 (7–9.5 Hz), alpha2 (9.5–12.5 Hz), beta1 (12.5–18 Hz), beta2 (18–30 Hz) and broadband (1.6–30 Hz) (Barnett, Seth, 2014).
Statistical data analysis. Linear regression analysis of coherence and GC indexes was performed for each pair of electrodes across every assessment of all children (146 EEG records) for girls and boys separately with the “age” as a continuous predictor. P levels below 0.01 and 0.001 (p < 0.01, p < 0.001) were considered as a significant estimation of linear regression (Statistica’10 software package). When analyzing patterns of the coherence and causality changes, we used the principle of finding “foci” of interaction, i.e. such electrodes (brain regions) that showed the connection with a large number of other zones (more than 3–5 connections) (Ivanitsky, 1997; Shemyakina, Danko, 2007). These regions could be considered as functional nodes of interactions.
RESULTS
Longitudinal EEG coherence changes
Regression analyses of EEG spectral coherence revealed a significant effect of the age factor in all frequency bands for separate analysis carried out in groupd of girls and boys. Due to the large number of statistical tests (120 electrode pairs for coherence and 240 for causality estimations), the significant level with the Bonferronni correction could be set as 0.0004 and 0.0002 for coherence and causality, respectively. This type of corrections could lead to type II errors, so we used a thresholding technique for correction. For the coherence estimation, the threshold was set at p < 0.001. This threshold reduced the number of electrode pairs for which changes were considered as significant from 100% to 33% in boys and 31% in girls. For those electrode pairs F(1, 57) – criteria values varied from 12 to 65, and betas varied from |0.41| to |0.73| in boys group; and F(1, 85) values varied from 11.7 to 34, betas values varied from |0.35| to |0.54| in girls group.
As seen in Fig. 1, the level of distant synchronization increased with age. Our results confirm the data that the strength of interregional synchronization is age-dependent (Schäfer et al., 2014). In the MEG study exploring the development of resting-state networks (including the FPN and DMN) between the ages of 6 and 34 years, there was reported a linear increase in connectivity in alpha and beta bands within these networks (Schäfer et al., 2014).
Fig. 1.
Longitudinal EEG coherence changes (as obtained by linear regression analysis) in groups of girls and boys aged 8 to 16–17 years. Notes: Red lines show age-related increases in EEG coherence values between the marked electrode locations. Differences were considered significant at p < 0.001 in regression analysis. EEG frequency bands: Delta (1.6–4 Hz), Theta (4–7 Hz), Alpha1 (7–9.5 Hz), Alpha2 (9.5–12.5 Hz), Beta1 (12.5–18 Hz), Beta2 (18–30 Hz), broadband (1.6–30 Hz).
Рис. 1. Возрастные изменения когерентности ЭЭГ (определенные методом линейного регрессионного анализа) в группах мальчиков и девочек. Примечание: Красная линия – увеличение когерентности между соответствующими электродами с возрастом. В качестве значимых рассматривали различия на уровне (p < 0.001). Диапазоны ЭЭГ: дельта (1.6–4 Гц), тета (4–7.5 Гц), альфа1 (7.5–9.5 Гц), альфа2 (9.5–12.5 Гц), бета1 (12.5–18 Гц), бета2 (18–30 Гц) и общем диапазоне частот ЭЭГ (1.6–30 Гц).
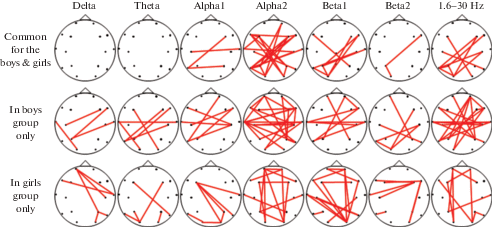
In our study, children and adolescences (girls and boys) demonstrated both age-related specific and common coherence maturation changes. Here we present first common and then specific changes in EEG coherence.
Age-related changes in coherence that are common for both genders were obtained mostly in alpha2 and broad bands. In alpha2 frequency band, coherence increased in the occipital region and in long-distance connections of the temporal and parietal areas of the left and right hemispheres with frontal areas of the contralateral hemisphere both in girls and boys. In broad and beta1 bands, common gender patterns were revealed between the left temporo-occipital areas and right frontal and central cortical areas.
Gender-specific age coherence changes demonstrated noticeable increases in connections of the right frontal and temporal areas with the left parieto-temporal and temporal areas (in delta, theta, alpha1, beta1, and broadband) in boys and between right occipital areas and left frontal, temporal areas (in alpha1 and beta1 frequency bands) in girls.
According to Fig. 1, there were a larger number of interhemispheric connections (especially in alpha2 and broad bands) in boys, while girls had a larger number of intrahemispheric long-distance connections between frontal and occipital areas with a noticeable age-related increase in alpha2 and broadband.
Longitudinal GC-indexes changes
Age-related changes in GC indices that could be considered as those of the information flow between the cortical areas were estimated by the linear regression analysis. For causality estimations, the threshold for the significant effect was set at p < 0.01. This threshold reduced the number of electrode pairs for which changes were considered as significant – from 100% to 36% in boys and to 34% in girls. With this p level in the boys group, F(1,55) values varied from 7.2 to 31 and betas varied from |0.34| to |0.6|; in the girls group, F(1,82) values varied from 7.2 to 18 and betas varied from |0.28| to |0.42|. Connections, for which there were obtained significant regression estimations with p < 0.01, are shown in Fig. 2.
Fig. 2.
Longitudinal changes in Granger causality indices (as obtained by linear regression analysis) in groups of girls and boys (8 and 16–17 years old). Red arrow indicates an increase in directed influence from one electrode location to another. Blue arrow indicates an age-related decrease in the influence of one zone on the other. EEG frequency bands: Delta (1.6–4 Hz), Theta (4–7 Hz), Alpha1 (7–9.5 Hz), Alpha2 (9.5–12.5 Hz), Beta1 (12.5–18 Hz), Beta2 (18–30 Hz) and broadband (1.6–30 Hz).
Рис. 2. Возрастные изменения причинности по Грейнджеру (определенные методом линейного регрессионного анализа) в группах мальчиков и девочек. Примечание: Красная линия – увеличение причинности (направление связи) между соответствующими электродами с возрастом, синяя линия – уменьшение причинности (направление связи) между соответствующими электродами с возрастом. В качестве значимых рассматривали различия на уровне (p < 0.01). Диапазоны ЭЭГ: дельта (1.6–4 Гц), тета (4–7.5 Гц), альфа1 (7.5–9.5 Гц), альфа2 (9.5–12.5 Гц), бета1 (12.5–18 Гц), бета2 (18–30 Гц) и общий диапазон частот ЭЭГ (1.6–30 Гц).
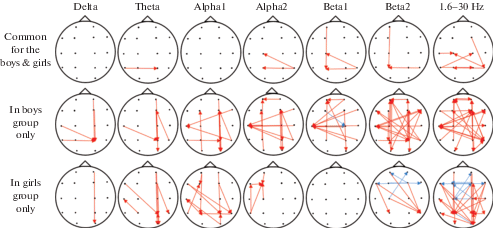
In both gender groups, there were observed increases in information flows from the right temporo-occipital area (T6) to the parietal and central areas of the left hemisphere. In broadband, changes in the causality indices were mostly symmetrical and related with the increases in the information flow from the right and left temporal-occipital areas toward the parietal and central areas of the contralateral hemispheres.
Age-related changes in the causality indices in boys included the bidirectional increase in the information flow between the occipital-parietal and frontal areas in the right hemisphere (in theta, apha1, and alpha2 bands), as well as from the occipital-parietal areas to the frontal areas in the left hemisphere (in alpha1, alpha2, beta1, and beta2 bands).
A distinctive feature of the boy group consisted in increases in information flows from the right hemisphere to the left temporal area (T3) in alpha, beta, and common bands. In the girl group, there was revealed a less number of causal directed flows from the posterior (parietal and occipital areas) to anterior (frontal area) brain sides. It demonstrates less influence of posterior brain cortex areas on frontal cortex areas with the age.
Information flows from the frontal to parietal and occipital areas, instead, increased in the theta, alpha1, beta2 and broad-frequency bands. The revealed decreases in the causality index in the central and frontal areas of both hemispheres (in beta2 and broadband) were a distinctive feature of the girls’ longitudinal development. In the alpha1 and alpha2 frequency bands, the hub gathering influences from the areas of the left hemisphere was situated in the left frontal region (F3).
Thus, the main feature distinguishing gender groups includes increases in the bidirectional information flow between the parietal and frontal regions in the boys group (mainly from the parietal to frontal areas) and increases in the information flow from the frontal to parietal areas in the girls group. The total number of connections changing with age in the girls group was smaller than in the boys group.
DISCUSSION
The developmental changes in EEG were analysed using longitudinal data of children living in the European North of Russia. We focused on age-related changes in the EEG coherence and causality indices. The longitudinal analysis suggests a reduction in the data variance, so that we could evaluate the changes in connectivity more clearly.
Spatial synchronization effects reflected by coherence. The obtained EEG coherence data demonstrated a well-pronounced age-related increase in the number of long-distance connections both in girls and boys, which is in agreement with the data on the interregional synchronization with age (Schäfer et al., 2014). Since we used an average montage (Ríos-Herrera et al., 2019), it was expected that the reduction in volume conductance could diminish the most number of short connections and coherence in low-frequency bands. At the same time, it was shown that long-distance synchronization increases during development, probably due to maturation and myelinization of long-distance association pathways (Barry et al., 2004; Gmehlin et al., 2011; Thatcher et al., 2008). Thatcher et al. (1986) proposed a two-compartmental model of cortico-cortical associations, in which short and long neuronal fibers contribute differentially to EEG coherence as a function of the inter-electrode distance. It was suggested that coherence between proximate electrodes mainly reflects the short white matter fibers (1–3 cm), whereas moderate to high coherence at long distances is supposed to reflect the long-distance white matter fasciculi (up to 25 cm). Human brain imaging studies revealed that the white matter increases through adolescence in cortical and subcortical fiber tracts (Benes et al., 1994; Paus et al., 1999, 2001; Asato et al., 2010), connected with increased myelination, axon caliber (Paus, 2010). The mostly linear increase in EEG coherence is in agreement with data on the morphofunctional brain development. As has been shown in contrast to the gray matter, the white matter volume increases in a roughly linear manner throughout the development till adulthood (Gogtay et al., 2004). These changes presumably reflect ongoing myelination of axons by oligodendrocytes that enhances neuronal conduction and communication (Casey et al., 2008). In our previous cross-sectional study, it was shown more prominent increases in coherence between old (14–17.9 y.o.) and medium (11–13.9 y.o.) age groups than between medium and young (7–10.9 y.o.) children groups (Soroko et al., 2015), indicating that functional connectivity formed continuously throughout the entire adolescence period (Tarokh et al., 2010). Thatcher et al. (Thatcher et al., 2008) described decreases in long-distance coherence and increases in short-distance coherence in a cross-sectional study of children and adolescents (0.44–16.22 y.o.). However, we considered a more focused time period of a longitudinal study (8–17 y.o.) and observed increases in short- and long-distance coherence probably related to the increase in connections through axons and fasciculi of the deep cerebral white matter (Schuz, Braitenberg, 2002).
In our study, coherence values increased in alpha2 and beta1 frequency bands, corresponding to common maturational trends, namely increasing the high-frequency EEG activity with the age (Farber et al., 1990, Segalowitz et al., 2010, Soroko et al., 2015b). On the other hand, a decrease in coherence during brain maturation could be associated with the differentiation of the activity of brain networks and the formation of the distributed system of autonomous and interconnected hubs (Fair et al., 2009).
The coherence alterations in alpha, as well as delta and theta, frequency bands were demonstrated in various developmental disorders, such as attention deficit/hyperactivity and autism (Barry et al., 2009; Coben et al., 2008), and could be indicators of the regulatory system maturity (Machinskaya et al., 2007).
An increase in functional coupling corresponds to the maturation of regulatory brain systems (Machinskaya, 2015) and their connectivity that ensures the development of cognitive abilities (Whedon et al., 2016; Machinskaya et al., 2019).
In our study, the common pattern of coherence increasement in the alpha-2 frequency band in boys and girls involved the lateral frontal areas (F7/F3, F8/F4) and temporo-parietal-occipital areas (T5/P3/O1, T6/P4/O2) of the contralateral hemisphere, which could be considered as local foci of interaction (Ivanitsky, 1997, Shemyakina, Danko, 2007). In terms of the networks it could be attributed to increase in functional connections between the left and right lateral frontoparietal networks (control network, includes dorsolateral prefrontal cortex and the anterior inferior parietal lobule), medial frontoparietal network (default network, includes temporoparietal junction) and occipital network (visual network, includes primary and secondary visual cortex) (Uddin et al., 2019).
In a fMRI study carried out by (Li et al., 2019), there were observed increased activation and connections of the dorsal attention network, default mode network, left frontoparietal network, and right frontoparietal network in “older” children group of early childhood (5-year-olds vs. 3-year-olds). It was suggested (Cui et al., 2020) that the energy required to activate the frontoparietal system declines with the age (across 8–23 y.o.), expecting executive functions and cognitive control enhancement.
The development of long-distance and interhemispheric connections through spectral coherence predominated in comparison with short distance connections in both genders (longitudinal study). The main gender-specific difference in EEG was attributed to an increase in the interhemispheric coherence in the alpha-2 frequency band in boys and an increase in alpha-2 frequency long-range anterior-posterior coherence in girls. From one point of view, the gender-specific differences in long-distant functional coupling could be associated with the variety in white matter maturation, which continues throughout the adolescent period (Kim et al., 2007, Herting et al., 2017; Vannucci et al., 2017) and might be influenced by endocrine changes in puberty (Herting et al., 2017), differently in boys and girls. However, there are also data showing no gender-specific effect on the maturation of white matter tracts (Genc et al., 2018). Different developmental trends in functional connectivity (coherence) are described in males and females (Barry, 2004), suggesting the developmental lag in the male group (Gmehlin et al., 2011, Campbell et al., 2012). In the case of northern conditions, gender-specific electrophysiological developmental differences might be more stressed/pronounced. The data on the pubertal (Tanner) stage in children demonstrated that northerner children (from the Arkhangelsk Region) had a 1–2-year lag in maturation and prolonged puberty compared to children living in more southern regions (Moscow Region) (Kubasov et al., 2004, Soroko et al., 2008). Since the pubertal lag was observed both in northerner boys and girls, the pubertal stage could be an important point for consideration in EEG maturation analysis in children from different regions. EEG spontaneous alpha activity was shown to be influenced by the pubertal stage and gender-specific (-related) differences (Howsley et al., 2018). The more advanced pubertal stage (late adolescence) predicted reduced EEG alpha power in males, but not females, that authors considered as the influence of testosterone on the cortical gray matter in males. In the other study (Feinberg et al., 2006), delta power decreases were age- and gender-modulated but not at the puberty stage. Since in our study we consider longitudinal changes for all adolescence period (4–5 Tanner stages in both – boys and girls) we use the age factor as a predictor for regression analysis. In our case, the fact that in boys during the considered period of observations (8–17 years) interhemispheric connections are intensely developing while in girls the same is true for anterior-posterior interrelations is debatable.
Longitudinal changes in GC-indices. Estimation of causality indices provides new information about directed functional (“causal”) interactions between cortical areas (Seth et al., 2015). The effective (directed) connectivity could be estimated in various data types (EEG, MEG, fMRI) by different methods, such as directed transfer function (DTF) (Kamiński et al., 2001, Baccala et al., 2016), partial directed coherence (PDC) (Baccala et al., 2001), transfer entropy methods (Barnett et al., 2009), and so on. We used a multivariate autoregression model based on GC (Barnett, Seth, 2014) to estimate linear causality in time series between electrodes.
We considered only those directed connections of longitudinal age-related changes, for which estimations were significant. The direction of connections was considered as the direction of the “information flow” from one cortical area to another (influence of one zone to another during development).
There were revealed the following several types of age-related changes: (1) A unidirectional age-related increase in the information flow, e.g., from temporo-occipital area of the right hemisphere to parietal and central areas of the left hemisphere in boys and girls in alpha2, beta1,2, and common-frequency bands. (2) A bidirectional age-related increase in the information flow between two regions, e.g., from the frontal to parietal areas of the right hemisphere (F3 → P3) and vice versa (P3 → F3) in alpha1,2 and beta2 frequency bands in boys. (3) A unidirectional age-related decrease in the information flow, e.g from frontal areas of the left and right hemispheres to the contralateral temporo-frontal areas (F3 → F8, F4 → F7) in beta2 and common frequency bands in girls. Thus, when considering GC index, it is possible to analyze changes in information flows in the large-scale brain systems during their development.
For example, the revealed common feature, an increase in the interhemispheric information flow from the temporo-occipital to parietal and central zones both in boys and girls, could be attributed to the maturation of the visual attention system (Uddin et al., 2019) and multimodal association brain areas.
The main differences observed between boys and girls related to the direction of the information flow between the anterior and posterior brain sides. In boys, the causal connections increased both from the frontal areas to the parietal and temporo-occipital areas of the right hemisphere (in theta, alpha1,2, beta2, and common frequency bands), and from the parietal, occipital and temporal areas of the left and right hemispheres to the frontal zones. By contrast, in girls, most causal connections demonstrated an increase in the information flow from the frontal to parietal areas. There was only one frontal zone (with the position at the F3 site) that was influenced by an increase in the information flow from other left-hemispheric areas in alpha1 and alpha2 frequency bands. The issue of whether all the observed gender-related differences were specific for northerners needs to be explored further.
GC indices support the relevance of the observed coherence changes for the development of fronto-parietal networks and highlight the maturation of the attention systems, reveal the directions of information flow increases during maturation.
Different directions of information flows in boys and girls during development might be pretended by different maturation rates of the regulatory systems, as well as bottom-up and top-down regulatory processes. As an example of visual attention processes by means of partial directed coherence, it was shown that the frontal regions can serve as influencing other areas sources in response to stimuli targets (top-down regulatory process), while the parietal regions serve as the main source of influence under non-target conditions (bottom-up processes) (Zhao, Wang, 2019).
Thus, we can suggest that the influence of the attention control system increases in the female group, while in males both processes developed with an increase in the influence of the posterior default mode network on the attention control system. From this point of view, it would make sense to consider individual characteristics of the participants, such as risk-taking and impulsivity that could differ between genders (Xu et al., 2020).
A combined consideration of data on functional and effective connectivity data may bring new information to understand the mechanisms of cognitive activity, development, and neurodegeneration (Anderson et al., 2010; Babiloni et al., 2016). For example, in a research by Anderson et al. (Anderson et al., 2010), an increase in functional connectivity (coherence) of cortical electrical activity between the prefrontal cortex and medial temporal lobe was revealed during recall from memory. In addition, GC demonstrated that the increased coherence referred to the higher bidirectional information flow between these regions (prefrontal and medial cortex), with a generally greater driving from the medial temporal lobe to the prefrontal cortex.
We can suggest an increase in information flows between cortical areas as the interaction index between and within the large-scale brain networks. According to our data was revealed an increase in interhemispheric coherence in boys and using causality analysis we can conclude that these coherent connections were mostly based on information flows from the right hemisphere to temporal zones of the left hemisphere. In girls, the long-distant anterior-posterior connections (by coherence analysis) referred to increases of information flows from frontal to parietal areas.
Thus, functional and effective connectivity, when studied as the complementary data, allowed tracking of the brain systems development during adolescence.
Study limitations. Longitudinal changes in functional and effective connectivity were estimated in populations living in the Russia’s northern regions. We assume that main patterns of children’s development are rather the same, regardless of the region of residence, while northern conditions can affect such parameters as the age of the formation of certain characteristic features. For example, when comparing the stages of the EEG structure formation, there were demonstrated the differences between the newcomer and indigenous populations of Northeast Russia (Soroko et al., 2012). There were no direct comparative longitudinal studies of children from the northern and more southern regions, but we can expect specificity of explored samples.
CONCLUSION
Brain maturation was accompanied by increases in functional and effective connectivity. Coherence (as functional coupling indices) increased mostly in alpha2, beta1, and broadband in interhemispheric anterior-posterior connections in both genders, corresponding, as we supposed, with interconnections between the fronto-parietal and visual attention systems and the development of information processes. The changes in causality suggest different trends of information flows changes with the age both in boys and girls. The bidirectional information flow increased between the posterior default mode network and the right fronto-parietal network in the boy group. By contrast, in girls, the information flow increased from the frontal to parietal areas in the central executive network (parietal network), suggesting the development of cognitive control.
Список литературы
Anderson K.L., Rajagovindan R., Ghacibeh G.A., Meador K.J., Ding M. Theta oscillations mediate interaction between prefrontal cortex and medial temporal lobe in human memory. Cereb Cortex. 2010. 20 (7): 1604–12. https://doi.org/10.1093/cercor/bhp223
Asato M.R., Terwilliger R., Woo J., Luna B. White matter development in adolescence: a DTI study. Cereb Cortex. 2010. 20 (9): 2122–2131. https://doi.org/10.1093/cercor/bhp282
Astolfi L., Cincotti F., Mattia D., Babiloni C., Carducci F., Basilisco A., Rossini P.M., Salinari S., Ding L., Ni Y., He B., Babiloni F. Assessing cortical functional connectivity by linear inverse estimation and directed transfer function: simulations and application to real data. Clin Neurophysiol. 2005. 116 (4): 920–932. https://doi.org/10.1016/j.clinph.2004.10.012
Babiloni C., Lizio R., Marzano N., Capotosto P., Soricelli A., Triggiani A.I., Cordone S., Gesualdo L., Del Percio C. Brain neural synchronization and functional coupling in Alzheimer’s disease as revealed by resting state EEG rhythms. Int J Psychophysiol. 2016. 103: 88–102. https://doi.org/10.1016/j.ijpsycho.2015.02.008
Baccalá L.A., Sameshima K. Partial directed coherence: A new concept in neural structure determination. Biol Cybern 2001. 84: 463–474.
Baccala L.A., Takahashi D.Y., Sameshima K. Directed Transfer Function: Unified Asymptotic Theory and Some of Its Implications. IEEE Trans Biomed Eng. 2016. 63(12):2450-2460. https://doi.org/10.1109/TBME.2016.2550199
Barnett L., Barrett A.B., Seth A.K. Granger causality and transfer entropy are equivalent for Gaussian variables. Phys Rev Lett. 2009. 103: 238701.
Barnett L., Seth A.K. The MVGC Multivariate Granger Causality Toolbox: A New Approach to Granger-causal Inference. J. Neurosci. Methods. 2014. 223: 50–68. https://doi.org/10.1016/j.jneumeth.2013.10.018
Barry R.J., Clarke A.R., McCarthy R., Selikowitz M. Adjusting EEG coherence for inter-electrode distance effects: an exploration in normal children. Int J Psychophysiol. 2005. 55 (3): 313–321. https://doi.org/10.1016/j.ijpsycho.2004.09.001
Barry R.J., Clarke A.R., McCarthy R., Selikowitz M. EEG coherence in children with attention-deficit/hyperactivity disorder and comorbid reading disabilities. Int J Psychophysiol. 2009. 71(3): 205–210. https://doi.org/10.1016/j.ijpsycho.2008.09.003
Barry R.J., Clarke A.R., McCarthy R., Selikowitz M., Johnstone S.J., Rushby J.A. Age and gender effects in EEG coherence: I. Developmental trends in normal children. Clin Neurophysiol. 2004. 115 (10): 2252–2258. https://doi.org/10.1016/j.clinph.2004.05.004
Bendat J.S., Piersol A.G. Random Data Analysis and Measurement Procedures. New York: Wiley. 1986.
Benes F.M., Turtle M., Khan Y., Farol P. Myelination of a key relay zone in the hippocampal formation occurs in the human brain during childhood, adolescence, and adulthood. Arch Gen Psychiatry. 1994. 51 (6): 477–484. https://doi.org/10.1001/archpsyc.1994.03950060041004
Bressler S.L., Seth A.K. Wiener Granger causality: a well established methodology. Neuroimage. 2011. 58 (2): 323–329.
Briley P.M., Liddle E.B., Groom M.J., Smith H.J.F., Morris P.G., Colclough G.L., Brookes M.J., Liddle P.F. Development of human electrophysiological brain networks. J Neurophysiol. 2018. 120 (6): 3122–3130. https://doi.org/10.1152/jn.00293.2018
Buzsáki G. Rhythms of the brain. Oxford University Press. 2006. 465 p.
Buzsáki G., Draguhn A. Neuronal oscillations in cortical networks. Science. 2004. 304(5679): 1926–1929. https://doi.org/10.1126/science.1099745
Campbell I.G., Grimm K.J., de Bie E., Feinberg I. Sex, puberty, and the timing of sleep EEG measured adolescent brain maturation. Proc Natl Acad Sci U S A. 2012. 109 (15): 5740–5743. https://doi.org/10.1073/pnas.1120860109
Casey B.J., Giedd J.N., Thomas K.M. Structural and functional brain development and its relation to cognitive development. Biol Psychol. 2000. 54 (1–3): 241–57. https://doi.org/10.1016/s0301-0511(00)00058-2
Casey B.J., Jones R.M., Hare T.A. The adolescent brain. Ann N Y Acad Sci. 2008. 1124: 111–126. https://doi.org/10.1196/annals.1440.010
Chorlian D.B., Tang Y., Rangaswamy M., O’Connor S., Rohrbaugh J., Taylor R., Porjesz B. Heritability of EEG coherence in a large sib-pair population. Biol Psychol. 2007. 75 (3): 260–266. https://doi.org/10.1016/j.biopsycho.2007.03.006
Coben R., Clarke A.R., Hudspeth W., Barry R.J. EEG power and coherence in autistic spectrum disorder. Clin Neurophysiol. 2008. 119 (5): 1002–1009. https://doi.org/10.1016/j.clinph.2008.01.013
Cohen D., Tsuchiya N. The Effect of Common Signals on Power, Coherence and Granger Causality: Theoretical Review, Simulations, and Empirical Analysis of Fruit Fly LFPs Data. Front Syst Neurosci. 2018. 12: 30. https://doi.org/10.3389/fnsys.2018.00030
Cui Z., Stiso J., Baum G.L., Kim J.Z., Roalf D.R., Betzel R.F., Gu S., Lu Z., Xia C.H., He X., Ciric R., Oathes D.J., Moore T.M., Shinohara R.T., Ruparel K., Davatzikos C., Pasqualetti F., Gur R.E., Gur R.C., Bassett D.S., Satterthwaite T.D. Optimization of energy state transition trajectory supports the development of executive function during youth. Elife. 2020. 9: e53060. https://doi.org/10.7554/eLife.53060
Dhamala M., Rangarajan G., Ding M. Analyzing information flow in brain networks with nonparametric Granger causality. Neuroimage. 2008. 41 (2): 354–362.
Fair D.A., Cohen A.L., Power J.D., Dosenbach N.U., Church J.A., Miezin F.M., Schlaggar B.L., Petersen S.E. Functional brain networks develop from a “local to distributed” organization. PLoS Comput Biol. 2009. 5 (5): e1000381. https://doi.org/10.1371/journal.pcbi.1000381
Farber D.A., Semenova L.K., Alferova V.V. Strukturno-funktsional’naya organizatsiya razvivayushchegosya mozga (Structural-Functional Organization of the Developing Brain). Leningrad: Nauka. 1990.
Feinberg I., Higgins L.M., Khaw W.Y., Campbell I.G. The adolescent decline of NREM delta, an indicator of brain maturation, is linked to age and sex but not to pubertal stage. Am J Physiol Regul Integr Comp Physiol. 2006 Dec;291 (6): R1724–9. https://doi.org/10.1152/ajpregu.00293.2006
Friston K.J. Functional and effective connectivity in neuroimaging: a synthesis. Hum. Brain Mapp. 1994. 2 (1–2): 56–78.
Genc S., Smith R.E., Malpas C.B., Anderson V., Nicholson J.M., Efron D., Sciberras E., Seal M.L., Silk T.J. Development of white matter fibre density and morphology over childhood: A longitudinal fixel-based analysis. Neuroimage. 2018. 183: 666–676. https://doi.org/10.1016/j.neuroimage.2018.08.043
Gevins A.S., Remond A. (Eds.). Handbook of Electroencephalography and Clinical Neurophysiology. Vol. 2: Methods and Analysis of Brain Electrical and Magnetic Signals. Amsterdam: Elsevier. 1987.
Geweke J. Measurement of linear dependence and feedback between multiple time series. J Am Stat Assoc. 1982. 77: 304–313.
Gmehlin D., Thomas C., Weisbrod M., Walther S., Resch F., Oelkers-Ax R. Development of brain synchronisation within school-age–individual analysis of resting (α) coherence in a longitudinal data set. Clin Neurophysiol. 2011. 122 (10): 1973–1983. https://doi.org/10.1016/j.clinph.2011.03.016
Gogtay N., Giedd J.N., Lusk L., Hayashi K.M., Greenstein D., Vaituzis A.C., Nugent T.F. 3rd, Herman D.H., Clasen L.S., Toga A.W., Rapoport J.L., Thompson P.M. Dynamic mapping of human cortical development during childhood through early adulthood. Proc Natl Acad Sci U S A. 2004. 101 (21): 8174–8179. https://doi.org/10.1073/pnas.0402680101
Gourevitch B., Le Bouquin-Jeannes R., Faucon G. Linear and nonlinear causality between signals: methods, examples and neurophysiological applications. Biol Cybern. 2006. 95 (4): 349–369.
Granger C.W.J. Investigating causal relations by econometric models and cross-spectral methods. Econometrica. 1969. 37 (3): 424–438.
Greicius M.D., Menon V. Default-mode activity during a passive sensory task: uncoupled from deactivation but impacting activation. Journal of Cognitive Neuroscience. 2004. 16: 1484–1492. PMID: https://doi.org/10.1162/ 089892904256853215601513
Gudmundsson S., Runarsson T.P., Sigurdsson S., Eiriksdottir G., Johnsen K. Reliability of quantitative EEG features. Clin. Neurophysiol. 2007. 118 (10): 2162–2171.
Herting M.M., Kim R., Uban K.A., Kan E., Binley A., Sowell E.R. Longitudinal changes in pubertal maturation and white matter microstructure. Psychoneuroendocrinology. 2017. 81: 70–79. https://doi.org/10.1016/j.psyneuen.2017.03.017
Howsley P., Levita L. Developmental changes in the cortical sources of spontaneous alpha throughout adolescence. Int J Psychophysiol. 2018 Nov; 133: 91–101. https://doi.org/10.1016/j.ijpsycho.2018.08.003
Hu S., Yao D., Bringas-Vega M.L., Qin Y., Valdes-Sosa P.A. The Statistics of EEG Unipolar References: Derivations and Properties. Brain Topogr. 2019. 32: 696–703. https://doi.org/10.1007/s10548-019-00706-y
Ivanitsky A.M. Informational synthesis in crucial cortical area as the brain base of the subjective experience. Zh. Vyssh. Nerv. Deiat. 1997. 47 (2): 223–225.
Kamiński M., Ding M., Truccolo W.A., Bressler S.L. Evaluating causal relations in neural systems: Granger causality, directed transfer function and statistical assessment of significance. Biol. Cybern. 2001. 85: 145–157.
Kim E.Y., Kim D.H., Yoo E., Park H.J., Golay X., Lee S.K., Kim D.J., Kim J., Kim D.I. Visualization of maturation of the corpus callosum during childhood and adolescence using T2 relaxometry. Int J Dev Neurosci. 2007. 25(6): 409–414. 2007.05.005.https://doi.org/10.1016/j.ijdevneu
Kozhushko N.J., Nagornova Z.V., Evdokimov S.A., Shemyakina N.V., Ponomarev V.A., Tereshchenko E.P., Kropotov J.D. Specificity of spontaneous EEG associated with different levels of cognitive and communicative dysfunctions in children. Int J Psychophysiol. 2018. 128: 22–30. https://doi.org/10.1016/j.ijpsycho.2018.03.013
Kubasov R.V., Demin D.B., Tipisova E.V., Tkachev A.V. Gormonal`noe obespechenie sistemoj gipofiz –shhitovidnaya zheleza – gonady` u mal`chikov v processe polovogo sozrevaniya, prozhivayushhix v Konoshskom rajone Arxangel`skoj oblasti [Hormonal supply of the system hypophysis – thyroid gland – gonads during puberty in boys living in the Konosha district of the Arkhangelsk region] (in Russ). Ekologiya cheloveka (Human ecology). 2004. 1 (S4): 265–268.
Kurth S., Achermann P., Rusterholz T., Lebourgeois M.K. Development of Brain EEG Connectivity across Early Childhood: Does Sleep Play a Role? Brain Sci. 2013. 3 (4): 1445–1460. https://doi.org/10.3390/brainsci3041445
Li C.L., Deng Y.J., He Y.H., Zhai H.C., Jia F.C. The development of brain functional connectivity networks revealed by resting-state functional magnetic resonance imaging. Neural Regen Res. 2019. 14 (8): 1419–1429. https://doi.org/10.4103/1673-5374.253526
Liao W., Mantini D., Zhang Z., Pan Z., Ding J., Gong Q., Yang Y., Chen H. Evaluating the effective connectivity of resting state networks using conditional Granger causality. Biol Cybern. 2010. 102 (1): 57–69.
Machinskaya R.I. The brain executive systems. Zhurnal vyssheĭ nervnoĭ deiatelnosti imeni I.P. Pavlova. 2015. 65 (1): 33–60.
Machinskaya R.I., Kurgansky A.V., Lomakin D.I. Age-related Trends in Functional Organization of Cortical Parts of Regulatory Brain Systems in Adolescents: an Analysis of Resting-State Networks in the EEG Source Space. Human Physiology. 2019. 45 (5): 461–473.
Machinskaya R.I., Sokolova L.S., Krupskaya E.V. Formation of the functional organization of the cerebral cortex at rest in young schoolchildren varying in the maturity of cerebral regulatory systems: II. Analysis of EEG α-rhythm coherence. Human physiology. 2007. 33 (2): 129–138.
Malekpour S., Li Z., Cheung B.L.P., Castillo E.M., Papanicolaou A.C., Kramer L.A., Fletcher J.M., Van Veen B.D. Interhemispheric effective and functional cortical connectivity signatures of spina bifida are consistent with callosal anomaly. Brain Connect. 2012. 2 (3): 142–154.
Nunez P.L., Silberstein R.B., Shi Z., Carpenter M.R., Srinivasan R., Tucker D.M., Doran S.M., Cadusch P.J., Wijesinghe R.S. EEG coherency II: experimental comparisons of multiple measures. Clin Neurophysiol. 1999. 110 (3): 469–486. https://doi.org/10.1016/s1388-2457(98)00043-1
Nunez P.L., Srinivasan R. Electric Fields of the Brain: The Neurophysics of EEG. Second Edition. New York: Oxford University press. 2006. 626 p.
Oldfield R.C. The assessment and analysis of handedness: the Edinburgh inventory. Neuropsychologia. 1971. 9 (1): 97–113. https://doi.org/10.1016/0028-3932(71)90067-4
Paus T. Growth of white matter in the adolescent brain: myelin or axon? Brain Cogn. 2010. 72 (1): 26–35. https://doi.org/10.1016/j.bandc.2009.06.002
Paus T., Collins D.L., Evans A.C., Leonard G., Pike B., Zijdenbos A. Maturation of white matter in the human brain: a review of magnetic resonance studies. Brain Res Bull. 2001. 54 (3): 255–266. https://doi.org/10.1016/s0361-9230(00)00434-2
Paus T., Zijdenbos A., Worsley K., Collins D.L., Blumenthal J., Giedd J.N., Rapoport J.L., Evans A.C. Structural maturation of neural pathways in children and adolescents: in vivo study. Science. 1999. 283 (5409): 1908–1911. https://doi.org/10.1126/science.283.5409.1908
Pereda E., Quian Quiroga R., Bhattacharya J. Nonlinear multivariate analysis of neurophysiological signals. Prog Neurobiol. 2005. 77: 1–37.
Raichle M.E., MacLeod A.M., Snyder A.Z., Powers W.J., Gusnard D.A., Shulman G.L. A default mode of brain function. Proceedings of the National Academy of Sciences of the United States of America. 2001. 98: 676–682. https://doi.org/10.1073/pnas.98.2.676
Ríos-Herrera W.A., Olguín-Rodríguez P.V., Arzate-Mena J.D., Corsi-Cabrera M., Escalona J., Marín-García A., Ramos-Loyo J., Rivera A.L., Rivera-López D., Zapata-Berruecos J.F., Müller M.F. The Influence of EEG References on the Analysis of Spatio-Temporal Interrelation Patterns. Front Neurosci. 2019. 13: 941. https://doi.org/10.3389/fnins.2019.00941
Ryali S., Supekar K., Chen T., Kochalka J., Cai W., Nicholas J., Padmanabhan A., Menon V. Temporal dynamics and developmental maturation of salience, default and central-executive network interactions revealed by variational bayes hidden markov modeling. PLoS Comput Biol. 2016. 12 (12): e1005138. https://doi.org/10.1371/journal.pcbi.1005138
Schäfer C.B., Morgan B.R., Ye A.X., Taylor M.J., Doesburg S.M. Oscillations, networks, and their development: MEG connectivity changes with age. Hum Brain Mapp. 2014. 35 (10): 5249–5261. https://doi.org/10.1002/hbm.22547
Schuz A., Braitenberg V. The human cortical white matter: Quantitative aspects of cortico-cortical long-range connectivity. In: Schultz A., Miller R., editors. Cortical Areas: Unity and Diversity, Conceptual Advances in Brain Research, London. Taylor and Francis, Inc., New York. 2002. 377–386.
Segalowitz S.J., Santesso D.L., Jetha M.K. Electrophysiological changes during adolescence: a review. Brain Cogn. 2010. 72: 86–100.
Seth A.K. A MATLAB toolbox for Granger causal connectivity analysis. J Neurosci Methods. 2010. 186 (2): 262–273. https://doi.org/10.1016/j.jneumeth.2009.11.020
Seth A.K., Barrett A.B., Barnett L. Granger causality analysis in neuroscience and neuroimaging. J Neurosci. 2015. 35 (8): 3293–3297. https://doi.org/10.1523/JNEUROSCI.4399-14.2015
Seth A.K., Edelman G.M. Distinguishing causal interactions in neural populations. Neural Comput. 2007. 19 (4): 910–933. https://doi.org/10.1162/neco.2007.19.4.910
Shemyakina N.V., Danko S.G. Changes in the power and coherence of the β2 EEG band in subjects performing creative tasks using emotionally significant and emotionally neutral words. Human Physiology. 2007. 33 (1): 20–26.
Singer W. Neuronal synchrony: a versatile code for the definition of relations? Neuron. 1999. 24 (1): 49–65, 111–125. https://doi.org/10.1016/s0896-6273(00)80821-1
Soroko S.I., Bekshaev S.S., Rozhkov V.P. [EEG correlates of geno-phenotypical features of the brain development in children of the native and newcomers' population of the Russian North-East] (in Russ). Ross. Fiziol. Zh. Im I. M. Sechenova. 2012. 98 (1): 3–26.
Soroko S.I., Bekshaev S.S., Rozhkov V.P., Nagornova Z.V., Shemyakina N.V. General features of the formation of EEG wave structure in children and adolescents living in Northern European Russia. Human Physiology. 2015b. 41 (4): 394–403. https://doi.org/10.1134/S0362119715040167
Soroko S.I., Burykh E.A., Bekshaev S.S., Rozhkov V.P., Sergeeva E.G. Khovanskikh A.E., Kormilitsyn B.N., Moralev S.N., Yagodina O.V., Dobrodeeva L.K., Maksimova I.A., Protasova O.V. Sravnitelnaya ocenka processa adaptacii u cheloveka v kontrastnyh ekologicheskih usloviyah (shirotnye effekty i posledstviya antropogennoj deyatelnosti). [Comparative assessment of the adaptation process in humans in contrasting environmental conditions (latitudinal effects and consequences of anthropogenic activity)]. pp. 182–204 in Izmenenie okruzhayushhej sredy i klimata: prirodnye i svyazannye s nimi texnogennye katastrofy. T. 4: Processy v biosfere: izmeneniya pochvenno-rastitel`nogo pokrova i territorial`ny`x vod RF, krugovorot veshhestv pod vliyaniem globalnyh izmenenij klimata i katastroficheskih processov. (Environmental and Climate Change: Natural and Related Technogenic Disasters. T. 4: Processes in the biosphere: changes in the soil and vegetation cover and territorial waters of the Russian Federation, the cycle of substances under the influence of global climate changes and catastrophic processes). (in Russ). G.A. Zavarzin, V.N. Kudeyarov (eds). – Pushchino; Moscow: IFX i BPP RAN, IFZ RAN. 2008. 206 p.
Soroko S.I., Nagornova Z.V., Rozhkov V.P., Shemyakina N.V. Age-specific characteristics of EEG coherence in children and adolescents living in the European North of Russia. Human Physiology. 2015. 41 (5): 517–531. https://doi.org/10.1134/S0362119715050151
Soroko S.I., Shemyakina N.V., Nagornova Zh.V., Bekshaev S.S. Longitudinal study of EEG frequency maturation and power changes in children on the Russian North. Int J Dev Neurosci. 2014. 38: 127–137. https://doi.org/10.1016/j.ijdevneu.2014.08.012
Tarokh L., Carskadon M.A., Achermann P. Developmental changes in brain connectivity assessed using the sleep EEG. Neuroscience. 2010. 171 (2): 622–634. https://doi.org/10.1016/j.neuroscience.2010.08.071
Thatcher R.W., North D.M., Biver C.J. Development of cortical connections as measured by EEG coherence and phase delays. Hum Brain Mapp. 2008. 29 (12): 1400–1415. https://doi.org/10.1002/hbm.20474
Tsitseroshin M.N., Ivonin A.A., Pogosyan A.A., Mikheev V.F., Galimov R.A. The role of the genotype in the development of the neurophysiological mechanisms involved in the spatial integration of neocortex bioelectric activity. Human Physiology. 2003. 29 (4): 393–407.
Uddin L.Q., Yeo B.T.T., Spreng R.N. Towards a Universal Taxonomy of Macro-scale Functional Human Brain Networks. Brain Topogr. 2019. 32 (6): 926–942. https://doi.org/10.1007/s10548-019-00744-6
van Baal G.C., Boomsma D.I., de Geus E.J. Longitudinal genetic analysis of EEG coherence in young twins. Behav Genet. 2001. 31 (6): 637–651. https://doi.org/10.1023/a:1013357714500
van Beijsterveldt C.E., Molenaar P.C., de Geus E.J., Boomsma D.I. Genetic and environmental influences on EEG coherence. Behav Genet. 1998. 28 (6): 443–453. https://doi.org/10.1023/a:1021637328512
Vannucci R.C., Barron T.F., Vannucci S.J. Development of the Corpus Callosum: An MRI Study. Dev Neurosci. 2017. 39 (1–4): 97–106. https://doi.org/10.1159/000453031
Whedon M., Perry N.B., Calkins S.D., Bell M.A. Changes in frontal EEG coherence across infancy predict cognitive abilities at age 3: The mediating role of attentional control. Dev Psychol. 2016. 52 (9): 1341–1352. https://doi.org/10.1037/dev0000149
Wiener N. The theory of prediction. Modern mathematics for the engineer. Ed. E. F. Beckenbach. McGraw-Hill. New York. 1956.
Xu W., Ying F., Luo Y., Zhang X.Y., Li Z. Cross-sectional exploration of brain functional connectivity in the triadic development model of adolescents. Brain Imaging Behav. 2020. https://doi.org/10.1007/s11682-020-00379-3
Zhao Z., Wang C. Using Partial Directed Coherence to Study Alpha-Band Effective Brain Networks during a Visuospatial Attention Task. Behav Neurol. 2019. 2019: 1410425. https://doi.org/10.1155/2019/1410425
Zhou Z., Chen Y., Ding M., Wright P., Lu Z., Liu Y. Analyzing brain networks with PCA and conditional granger causality. Hum Brain Mapp. 2009. 30: 2197–2206. https://doi.org/10.1002/hbm.20661
Дополнительные материалы отсутствуют.
Инструменты
Журнал высшей нервной деятельности им. И.П. Павлова