Почвоведение, 2023, № 11, стр. 1357-1370
Vis-NIR спектроскопия для целей оценки содержания органического углерода почв (метаанализ)
А. В. Чинилин a, *, Г. В. Виндекер a, И. Ю. Савин a, b
a Почвенный институт им. В.В. Докучаева
119017 Москва, Пыжевский пер., 7, стр. 2, Россия
b Российский университет дружбы народов, Институт экологии
115093 Москва, Подольское ш., 8, стр. 5, Россия
* E-mail: chinilin_av@esoil.ru
Поступила в редакцию 10.04.2023
После доработки 19.06.2023
Принята к публикации 21.06.2023
- EDN: TOPMFN
- DOI: 10.31857/S0032180X23600695
Аннотация
Выполнен обзор и метаанализ научных статей, посвященных оценке содержания органического углерода почв с применением подходов Vis-NIR спектроскопии. В обзор вошло 134 исследования, опубликованных в период с 1986 по 2022 гг. с общей выборкой в 709 значений количественных метрик. Поиск статей проводили в научных поисковых системах: РИНЦ, Science Direct, Scopus, Google Scholar по ключевым словосочетаниям: “спектроскопия почв” и “Vis-NIR spectroscopy AND soil organic carbon”. В процессе метаанализа при помощи непараметрического одностороннего дисперсионного анализа Краскела–Уоллиса в совокупности с непараметрическим методом попарного сравнения выполняли определение наличия статистически значимой разницы между медианными значениями принятых количественных метрик предсказательной силы моделей (коэффициента детерминации ($R_{{{{{\text{cv}}} \mathord{\left/ {\vphantom {{{\text{cv}}} {{\text{val}}}}} \right. \kern-0em} {{\text{val}}}}}}^{2}$), корня среднеквадратичной оценки (RMSE) и отношения производительности к отклонению (performance to deviation, RPD)). В результате выявлена наилучшая эффективность метода предварительной обработки спектральных кривых. Проведено сравнение результатов оценки содержания органического углерода почв между методом спектроскопии в лаборатории и в полевых условиях.
ВВЕДЕНИЕ
Для количественной оценки изменений в содержании и составе органического углерода почв, которые могут возникнуть в результате изменения характера землепользования, смены приемов обработки почв, необходимы практичные и бюджетные методы измерения и мониторинга. Стандартные аналитические процедуры могут быть чрезвычайно трудоемкими и дорогостоящими, особенно в случае большого количества образцов при потоковых анализах [49]. Достижения в области бесконтактного почвенного зондирования, в частности спектроскопии почв, а также успехи в области приборостроения могут быть использованы для целей оценки свойств почв [71].
Спектроскопия почв (как в лаборатории, так и полевая) рассматривается как альтернатива (не замена, но дополнение), направленная на улучшение традиционных методов анализа свойств почв, “сухая химия”. Это быстрый, точный, безвредный для окружающей среды, воспроизводимый и экономически эффективный аналитический метод бесконтактной оценки свойств почв [35, 46, 69]. Спектроскопия почв также может выступать в качестве базы для дальнейшей идентификации и распознавания почв с помощью спутниковой информации: модели для начала калибруются с помощью данных лабораторной или полевой спектрометрии, затем по полученным наиболее информативным длинам волн осуществляется переход к спутниковой информации (в идеальном случае к гиперспектральным данным) и получают картографические модели, отражающие пространственную дифференциацию интересующих свойств почв на уровне поля. Такой синергетический анализ получил название “снизу–вверх” [16, 17].
Спектральные особенности почв детально изучались в период освоения аэрометодов в почвоведении, начиная с 1950-х гг. Андрониковым [1], Ливеровским [6], Толчельниковым [11], Кармановым [3, 4]. Установлено, что различные почвы, минералы, виды растительности по-разному отражают, поглощают и излучают электромагнитные волны различных областей спектра, следовательно, каждая почва имеет свой спектральный “отпечаток” в зависимости от состава и состояния. Приблизительно в тот же период под руководством Кононовой [5] апробирован метод спектроскопии в ультрафиолетовой и видимой областях спектра, что позволило выявить характерные особенности гумусообразования почв различных почвенных зон. Орловым [8] разработана классификация спектров отражения большинства почв и их генетических горизонтов. За рубежом первые работы в области почвенной спектроскопии относятся к 1970–1980-м гг. [23, 83]. Прошло еще 30 лет до тех пор, как спектроскопия почв стала широко востребованным направлением [41].
Среди спектроскопических методов рассматривают, главным образом, спектроскопию в видимой-ближней инфракрасной (Vis-NIR), инфракрасной (NIR) областях спектра, спектроскопию в среднем диапазоне длин волн (MIR), которая включает спектроскопию с преобразованием Фурье (FTIR) и Рамановскую спектроскопию.
Согласно научной литературе, использование MIR-спектроскопии (2500–25 000 нм или 4000–400 см–1) для оценки и прогнозирования свойств почв превосходит спектроскопию в видимой-ближней инфракрасной области спектра [12, 54, 60], что связано с более четко выраженными фундаментальными полосами поглощения химических связей (N–H, C–H, C–O) в среднем диапазоне длин волн в результате валентных и деформационных колебаний [62]. Происхождение полос поглощения в этой области спектра связано с колебаниями отдельных атомов или атомных групп, и вращением молекулы в целом. Полосы поглощения в Vis-NIR области спектра являются результатом обертонов (и их комбинаций) вышеназванных колебаний, выражены не так ярко (меньше и шире), перекрываются друг с другом и менее различимы. Так, к примеру, органическое вещество почв имеет слабую полосу поглощения в области 1650–1700 нм (NIR), что обусловлено первым обертоном деформационных основных (фундаментальных) колебаний метильных (–CH2) и метиленовых (=CH2) групп в области 3400 нм (MIR). Однако MIR-спектроскопия практически не реализована в виде портативных приборов, которые могут применяться в полевых условиях. В пользу использования Vis-NIR спектроскопии говорит и меньшая стоимость данной технологии, что делает ее более доступной.
Применительно к анализу почв наибольший интерес представляет спектроскопия диффузного рассеяния (diffuse reflectance spectroscopy), так как при рассеянном отражении энергия электромагнитного спектра проникает и взаимодействует с физическими и химическими составляющими почвы, при этом глубина проникновения прямо пропорциональна длине волны. Спектроскопия диффузного рассеяния используется для регистрации спектров гетерогенных систем, порошков или твердых веществ, при этом диффузно отраженное от образца излучение собирается под широким углом и передается на детектор, в отличие от традиционно используемой спектроскопии пропускания, где для регистрации спектров используют кюветы, прессование образца, стекла.
Vis-NIR (400–2500 нм или 25 000–4000 см–1) спектроскопия диффузного рассеяния с успехом используется для прогнозирования широкого ряда физических и химических свойств почв: цвета [51], гранулометрического состава [22, 27, 28], влажности [10, 43, 57], содержания органического [26, 59, 63] и неорганического [29, 31] углерода почв, пулов и фракций органического углерода в почве [33, 61, 69, 76], обменных оснований [52, 73], pH [59, 75], засоления [36, 82], минералогического состава [40, 68], различных форм железа [67, 78], содержания тяжелых металлов и других загрязняющих веществ [25, 47]. Vis-NIR спектроскопия дополняет молекулярные подходы к характеристике разнообразия и биогеографии почвенных грибов и микробных сообществ [79, 80]. Оценке и прогнозированию поддаются свойства почв, как напрямую влияющие на спектр электромагнитного излучения (органические и неорганические соединения углерода, минералогический состав, оксиды железа), так и свойства (pH, состав обменных катионов, емкость катионного обмена), не вызывающие активного спектрального поглощения, но имеющие корреляцию со спектрально активными свойствами.
Будучи одним из важнейших компонентов, играющих роль в формировании окраски почв, органический углерод почв существенно влияет на форму и характер спектров отражения и обладает очевидными спектральными особенностями [7, 8]. Однако задача интерпретации зарегистрированного спектрального сигнала в отношении органического углерода почв не является тривиальной из-за сложности состава его соединений, перекрытия полос поглощения другими компонентами матрицы почв [62]. Поэтому для количественного анализа требуются калибровочные модели (в совокупности с предварительной обработкой спектральных кривых), извлекающие из спектров сложные схемы поглощения, генерируемые почвенными составляющими.
МЕТОДОЛОГИЯ
Метаанализ – распространенный и эффективный инструмент анализа научных публикаций, который представляет собой процедуру, суммирующую данные из ряда работ и делающую на их основе общий статистический вывод.
Обзор и метаанализ в этом исследовании включают в себя следующие шаги: определение цели исследования, поиск научных статей на основе ключевых слов с последующим извлечением данных, исключение не релевантных исследований или дублирующихся статей, формальный анализ.
Поиск статей проводился в научных поисковых системах: Science Direct, Scopus, Google Scholar по ключевым словосочетаниям “Vis-NIR spectroscopy AND soil organic carbon”. Поиск также осуществлялся по базе РИНЦ по словосочетанию “спектроскопия почв”. Часть статей исключалась, исходя из их названия, часть – путем анализа аннотации в связи с несоответствием тематике. В результате из оставшихся 134 работ (опубликованных в период с 1986 по 2022 гг.) извлекались данные для проведения метаанализа.
В статьях, включенных в метаанализ, используются подходы Vis-NIR спектроскопии образцов почв и последующее сравнение результатов со стандартными лабораторными процедурами с помощью принятых количественных метрик предсказательной силы модели. В качестве метрик извлекались следующие значения: коэффициент детерминации ($R_{{{\text{cv/val}}}}^{2}$), корень среднеквадратической оценки (RMSE) и отношение производительности к отклонению (performance to deviation, RPD). В то время как более высокие значения коэффициента детерминации и RPD представляют лучшие прогнозы, более низкие значения RMSE указывают на более высокую точность. Коэффициенты уравнения регрессии, показывающие силу и характер влияния независимых переменных на зависимую и характеризующие степень значимости отдельных переменных, нами не извлекались, так как приводятся авторами в единичных исследованиях.
Для определения наличия статистически значимой разницы между медианами трех или более независимых групп значений использовался непараметрический односторонний дисперсионный анализ Краскела–Уоллиса (критерий Уилкоксона–Манна–Уитни в случае двух групп) в совокупности с непараметрическим методом попарного сравнения (апостериорный критерий Данна). Для визуализации результатов использовалась свободная программная среда вычислений R и пакет “ggstatsplot” [48].
РЕЗУЛЬТАТЫ И ОБСУЖДЕНИЕ
Количество публикаций по теме использования Vis-NIR спектроскопии для целей оценки и содержания органического углерода почв заметно увеличилось за последние несколько лет. Так, из статей, включенных в метаанализ, за период с 2018 по 2022 гг. опубликовано 66 научных работ, что составляет 49% от общего числа публикаций, что может быть связано с необходимостью бюджетного и практичного метода измерения свойств почв, а также с все большим вниманием и интересом к данному подходу научного сообщества.
В большинстве (84%) проанализированных работ подходы спектроскопии почв апробировались в лабораторных условиях, оставшиеся 16% использовали измерения in situ или с помощью передвижных платформ (on-the-go).
Проанализированные исследования охватывают масштабные уровни от поля до континентальных и глобальных оценок содержания органического углерода почв.
Предварительная обработка спектральных кривых. Обычно спектральные кривые отражения похожи, и по ним далеко не всегда удается выделить особенности тех или других почв [8]. Выбор надежного способа предварительной обработки спектральных кривых может помочь в построении более точной модели прогнозирования исследуемого свойства. Это первый и важный шаг анализа спектральных данных. Различные способы предобработки исходных спектральных данных используются для уменьшения шума и артефактов, улучшения отношения сигнала к шуму, улучшения характеристик поглощения, уменьшения эффекта рассеяния света, уменьшения размерности исходных необработанных или “сырых” спектров. Наиболее часто используемыми методами предварительной обработки спектральных данных являются [15, 72]: фильтрация скользящим средним (MA), сглаживание Савицкого–Голея (SG), расчет первой производной (FD), мультипликативная коррекция рассеяния (MSC), нормализация спектра (SNV), вэйвлет-трансформация (WT), удаление континуума (CR), преобразование значений отражения (R) в значения поглощения (A = lg(1/R)), удаление линейного тренда из спектра (DT). В ряде работ исследователи предпочли использовать комбинацию нескольких методов предварительной обработки: фильтрацию Савицкого-Голея с расчетом первой производной (SGD), фильтрацию Савицкого–Голея и преобразование в значения поглощения (A*), нормализацию SNV и преобразование в значения поглощения (A**), в то время некоторые исследования прямо утверждают, что предварительная обработка спектров не улучшает прогноз [45]. На сегодняшний день не существует “золотого стандарта” по использованию методов предобработки (или последовательности методов), поэтому предпринимаются подходы опробования нескольких из них и выбора лучшего [21, 24, 44]. Из 134 проанализированных публикаций 14 (≈10%) относится к исследованиям, направленным на изучение влияния различных методов предварительной обработки. На рис. 1 показаны результаты применения нескольких методов предварительной обработки спектральных данных.
Рис. 1.
Примеры спектров отражения в Vis-NIR диапазоне с различными методами предварительной обработки данных: (a) исходные необработанные спектры (R), (b) спектры поглощения (A), (c) спектры после фильтрации Савицкого–Голея (SG), (d) спектры первой производной (FD), (e) спектры после стандартного нормального изменения (SNV), (f) спектры с удалением линейного тренда (DT). Исходные спектры взяты из открытой спектральной библиотеки ICRAF-ISRIC Soil VNIR Spectral Library. Предобработка проведена с помощью программной среды вычислений R и пакета “prospectr” [64].
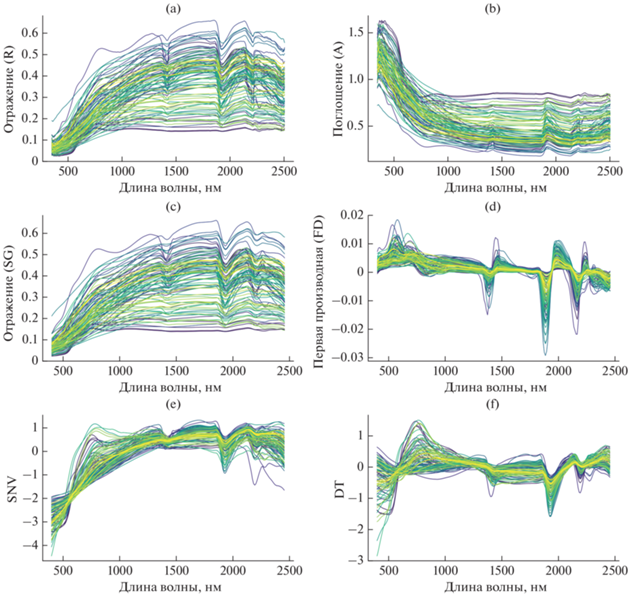
На рис. 2 показано сравнение медианных значений коэффициента детерминации и ошибки прогноза (RMSE) для различных используемых методов предварительной обработки спектральных кривых. Так, лучшую эффективность (с точки зрения коэффициента детерминации) показывают следующие методы (рис. 2a): вейвлет-трансформация (WT) с R2 = 0.81, фильтрация Савицкого–Голея с преобразованием в значения поглощения (A*) с R2 = 0.78, фильтрация Савицкого–Голея (SG) с R2 = 0.77, первая производная (FD) с R2 = 0.74.
Рис. 2.
Сравнение медианных значений коэффициента детерминации (R2) и ошибки прогноза (RMSE) для различных используемых методов предварительной обработки спектральных кривых. Горизонтальными линиями отражены значимо отличающиеся медианы групп значений. Аббревиатуры расшифрованы по тексту.
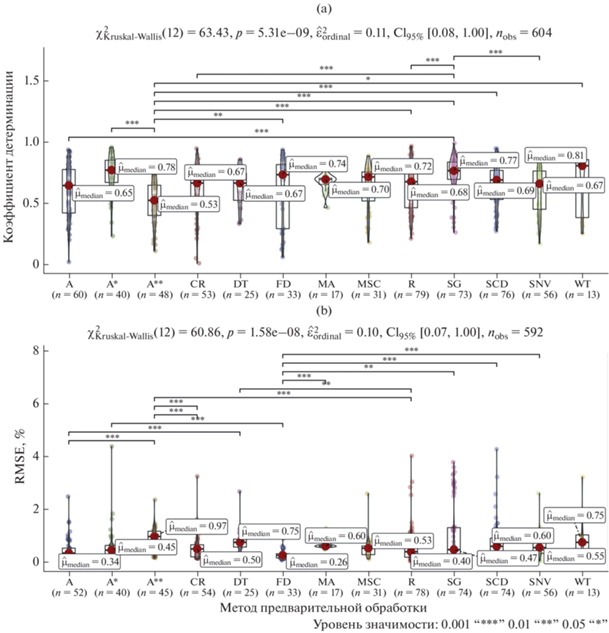
Вейвлет-преобразование (WT) представляется полезным инструментом для обработки спектров с точки зрения фильтрации и уменьшения размерности (сжатия данных). WT идеально подходит для извлечения спектральных признаков, прежде всего потому, что выполняет много-масштабный анализ сигнала [37], дальнейший анализ на разных масштабах (или разрешениях) может улучшить идентификацию различных спектральных особенностей [58]. Фильтрация Савицкого–Голея (SG) широко используется для предобработки спектров почв применительно и к MIR-спектроскопии. Этот способ универсален и подходит для сглаживания, фильтрации, шумоподавления. Математически он работает как взвешенная сумма соседних значений. Подход имеет несколько параметров тонкой настройки: размер окна сглаживания, параметр дифференцирования, порядок полиномов, определяющих степень фильтрации [56]. Взятие первой производной (FD) устраняет аддитивные и мультипликативные (например, размер частиц) эффекты в спектрах [72]. К плюсам этого метода можно отнести следующее: устранение перекрытия участков поглощения, усиление слабых участков поглощения, устранение инструментального дрейфа. К недостаткам: увеличение шума, следовательно требуется сглаживание, усложнение интерпретации спектра, риск “переобучения” калибровочной модели [30]. Поэтому следует быть осторожным при использовании этого подхода предобработки, так как калибровочную модель можно исказить, генерируя связь с шумом, а не с реальным почвенным свойством.
Метаанализ показывает, что почти в каждом десятом исследовании авторы используют либо фильтрацию Савицкого–Голея (SG), либо комбинацию фильтрации Савицкого–Голея с расчетом первой производной (SGD).
Наименьшую ошибку прогноза при оценке содержания органического углерода почв с помощью Vis-NIR спектроскопии обеспечивают (рис. 2b): первая производная (FD) RMSE = 0.26, спектры поглощения (A) RMSE = 0.34, исходные спектры (R) RMSE = 0.40.
Отметим, что исходные необработанные спектры (R) дают лучшие результаты производительности моделей по сравнению с рядом подходов предобработки, что подтверждает гипотезу о необходимости итеративного эвристического анализа спектральных данных. Предположение в том, что применительно к различным почвенно-биоклиматическим условиям нужен свой подход опробования нескольких способов предварительной обработки и выбора того, который существенно улучшает производительность модели путем вычленения и идентификации характерных полос поглощения для почв с различным содержанием органического углерода. Можно предположить, что определенная предварительная обработка устраняет влияние некоторых компонентов почвы, что повышает чувствительность к оценке содержания органического углерода почв. Наиболее подходящие методы будут зависеть от варьирования оцениваемого свойства, качества исходных данных, погрешностей спектрорадиометра, условий съемки, метода многомерного анализа.
Перечень способов предварительной обработки не исчерпывается выше перечисленными. Существуют узконаправленные методы первоначальной обработки спектральных данных, к примеру, коррекция “склейки” (splice correction). Последний применяется для устранения погрешностей при съемке с помощью спектрорадиометров ASD FieldSpec Pro (Malvern Panalytical, Malvern, Worcestershire, United Kingdom), где наблюдается переход между различными детекторами. Эти переходы обычно происходят при длинах волн в 1000 и 1830 нм [64]. Например, метод Гауссовых пирамид (широко используется и при анализе растровых изображений), который представляет собой иерархический подход сжатия данных, подобный вейвлет-трансформации [13]. В качестве эффективного способа смягчения влияния влажности почв предложен подход ортогонализации внешних параметров (external parameter orthogonalization), разделяющий спектр на “полезную” часть, содержащую характеристики почв, и “внешнюю” часть, подверженную влиянию влаги [21, 32, 53]. Однако подобные способы используются не так часто (и даже единично) и оказываются за рамками настоящего метаанализа.
Многомерный анализ данных. Такие факторы, как перекрывающиеся полосы поглощения почвенных составляющих, инструментальный шум, эффект рассеяния приводят к сложным схемам поглощения, и задача при сборе данных заключается в математическом извлечении из спектров информации, которая коррелирует со свойствами почв. Данная задача относится к области дисциплины хемометрики, находящейся на стыке химии и математики и применяющей математические и статистические методы для построения оптимальных методов измерения, а также для извлечения наиболее важной информации при анализе экспериментальных данных [9, 38].
Наиболее распространенными способами калибровки моделей связи между спектральными характеристиками (x1, x2…, xn) и свойствами почв (y) являются [72]: множественная линейная регрессия (MLR), регрессия на главные компоненты (PCR), регрессия частично наименьших квадратов или метод проекции на латентные структуры (PLSR), искусственные нейронные сети (NN), ансамбль деревьев решений (RF), метод опорных векторов (SVM), модель правил регрессии (Cubist), подходы локального моделирования (MBL), схожие по принципу с подходом k-ближайших соседей. Из 134 проанализированных публикаций в 107 (≈80%) авторы используют регрессию частично наименьших квадратов.
Точность прогнозирования содержания органического углерода почв с помощью Vis-NIR спектроскопии проиллюстрирована на рис. 3 (вне зависимости от метода многомерного анализа и способа предобработки). Медианное значение коэффициента детерминации (R2) по всем исследованиям составляет 0.67, ошибки прогноза – 0.48, RPD – 1.99. Согласно шкале Р.Е. Чеддока, а также [70], когда RPD принимает значения меньше 1.5 и коэффициент детерминации меньше 0.5 — прогнозы неудавшиеся; значения RPD от 1.5 до 2 и значения R2 от 0.5 до 0.7 — прогнозы посредственные (но с возможностью разделения высоких и низких значений); значения RPD от 2 до 2.5 и значения R2 от 0.7 до 0.9 – прогнозы достаточной точности с хорошей количественной оценкой; значения RPD выше 2.5 и значения R2 выше 0.9 – отличные прогнозы.
Рис. 3.
Точность прогнозирования содержания органического углерода почв с помощью Vis-NIR спектроскопии. Цвета от красного до зеленого представляют неудачные, умеренные, хорошие и отличные прогнозы, соответственно. Дополнительно, по осям представлены гистограммы распределения значений коэффициента детерминации (R2) и RPD.
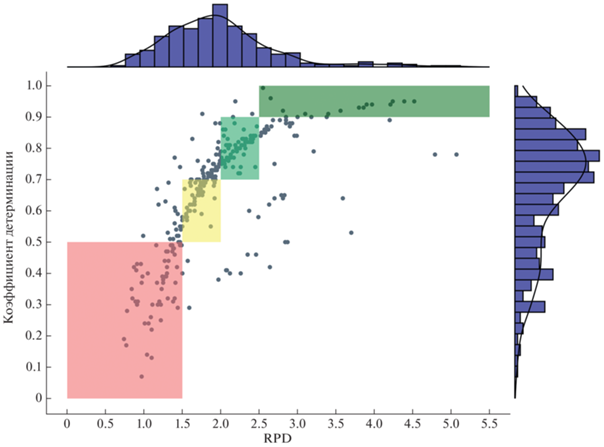
Рис. 3 (гистограммы распределения, в частности) показывает, что большинство прогнозов лежит в области умеренных и хороших оценок. Одно из главных критических замечаний, относящихся к спектроскопии почв, состоит в том, что она не показывает столь точные результаты по сравнению с традиционными лабораторными способами [39]. Однако традиционные лабораторные способы фактически являются стандартами и любые другие методы, направленные на получение тех же результатов, неизбежно являются менее точными, поскольку они основаны на калибровке спектральных данных по стандартам и допускают статистическую погрешность. Плюс Vis-NIR спектроскопии в том, что при равных затратах можно провести на порядок больше измерений, таким образом дисперсия оценки будет меньшей по сравнению с обычным лабораторным анализом. Большее количество измерений также позволит снизить влияние случайных погрешностей.
На рис. 4 показано сравнение медианных значений коэффициента детерминации и ошибки прогноза (RMSE) для различных используемых многомерных подходов анализа данных. Лучшую эффективность (с точки зрения коэффициента детерминации) показывают следующие методы (рис. 4a): нейронные сети (NN) с R2 = 0.77, множественная линейная регрессия (MLR) с R2 = 0.71, регрессия на главные компоненты (PCR) с R2 = 0.70, регрессия частично наименьших квадратов (PLSR) с R2 = 0.69. Два последних метода широко используются в хемометрике для количественного анализа спектров диффузного рассеяния. Оба метода используются для построения калибровочных моделей, когда имеется большое количество независимых переменных (предикторов), которые сильно коллинеарны. Оба метода справляются с “проклятием размерности” и сжимают данные перед выполнением регрессионного анализа на ряд ортогональных переменных (главные компоненты в случае PCR, скрытые переменные в случае PLSR). Отличие подходов лежит в построении новых переменных. Для PCR неважно, как переменные-предикторы связаны с зависимой переменной. В отличие от PCR, алгоритм PLSR объединяет этапы сжатия и регрессии и выбирает последовательные ортогональные переменные, которые максимизируют ковариацию между переменными-предикторами (x1, x2…, xn) и откликом (y).
Рис. 4.
Сравнение медианных значений коэффициента детерминации (R2) и ошибки прогноза (RMSE) для различных используемых подходов многомерного анализа данных. Горизонтальными линиями отражены значимо отличающиеся медианы групп значений. Аббревиатуры расшифрованы по тексту.
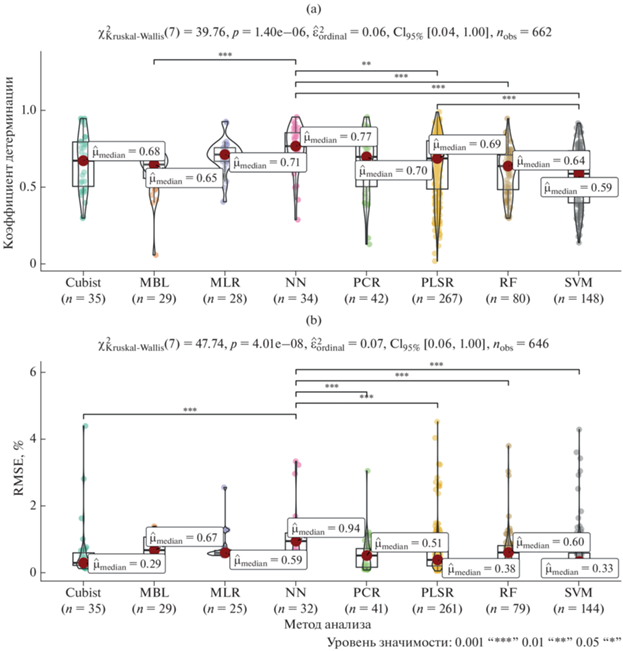
Нейронные сети (NN) – мощный метод моделирования, позволяющий воспроизводить чрезвычайно сложные зависимости, в особенности нелинейные. Это особенно актуально для задач, где линейная аппроксимация (MLR) работает неудовлетворительно. Кроме того, нейронные сети справляются с сокращением размерности. Однако этот метод не всегда позволяет понять, на основании чего та или иная модель совершает прогноз (проблема черного ящика), и сделать на основании этого обоснованные выводы, что может подрывать доверие к таким моделям. Хотя в настоящее время используются подходы по интерпретации сложных моделей (к примеру, вектор Шепли) с целью отразить влияние отдельных переменных на результат [42, 81].
Наименьшую ошибку прогноза при оценке содержания органического углерода почв с помощью Vis-NIR спектроскопии обеспечивают (рис. 4b): модель правил регрессии (Cubist) RMSE = 0.29, машина опорных векторов (SVM) RMSE = 0.33, регрессия частично наименьших квадратов (PLSR) RMSE = 0.38. Первый из методов очень популярен в настоящее время и является легко интерпретируемым и объяснимым. Этот метод использует условия вида “если, то” для разделения спектральных данных на взаимосвязанные ветви и аппроксимирует хорошо понятные модели линейной регрессии к результатам каждого разделения [34].
Бо́льшая ошибка может быть связана с тем, что в исследованиях регионального, национального и глобального масштабов в калибровочные и тестовые подмножества попадают почвы, значительно отличающиеся по свойствам. Например, в калибровочную подвыборку в большей мере попадают гумусово-гидрометаморфические и аллювиальные темногумусовые почвы с содержанием органического углерода 6–9%, а в тестовую подвыборку – черноземы текстурно-карбонатные с содержанием органического углерода 3–4%. Одно из возможных решений – построение моделей на определенном таксономическом уровне, т.е. на уровне типа. Другое возможное решение – исследование методов выбора подмножеств для обучения и валидации модели (кластерный анализ), оценка репрезентативности данных, отбраковка “выбросов”.
В последнее время значительные усилия направлены на разработку и оценку различных процедур ранжирования, которые объективно идентифицируют спектральные переменные, вносящие больший вклад, и одновременно устраняют неинформативные переменные, вносящие шум в модель. Это позволяет упростить модель, избежать переобучения, повысить интерпретируемость. Процедуры ранжирования включают некоторые классические подходы, такие как экспертные знания, рекурсивное исключение признаков, а также более сложные: генетический алгоритм, регуляризованные деревья, алгоритм оптимизации подражанием муравьиной колонии [77].
Нельзя не отметить и тот факт, что исходное варьирование содержания органического углерода почв на различных уровнях масштаба во многом определяет точность моделей оценки. На уровне национальных и глобальных спектральных библиотек почв, содержащих тысячи наблюдений, отмечается большее варьирование содержания органического углерода почв, и, как следствие, – большее смещение (математическое ожидание разности между истинными и прогнозируемыми значениями) в результирующих моделях. В региональном масштабе один и тот же тип почв может характеризоваться различным содержанием органического углерода из-за фациальных особенностей, эрозионных процессов, выпаханности и отличаться спектрами отражения. С другой стороны, на уровне поля почвы с близким содержанием органического углерода, но развитые на различных почвообразующих породах, также будут отличаться по спектральному облику. Объединение региональных данных с множеством локальных в спектральные библиотеки национального масштаба и получение общих моделей дает предвзятые результаты. Полученные общие взаимосвязи по таким моделям не дают представления о локальных изменениях в содержании органического углерода почв. В настоящее время становятся популярными подходы “дополнения” (spiking), например, региональных выборок с ограниченным числом данных (спектрально и географически наиболее похожих) из локальных выборок [66].
Лабораторная спектроскопия versus спектроскопии in situ. На рис. 5 показано сравнение результатов оценки содержания органического углерода почв между спектроскопией в лаборатории (график справа) и непосредственно в полевых условиях. Лучшую эффективность (с точки зрения коэффициента детерминации) показывают результаты лабораторной спектроскопии, R2 выше на 20% по сравнению со спектроскопией in situ (рис. 5a). Однако лабораторная спектроскопия показывает и большую неопределенность, на что указывают большие интервалы значений ошибки прогноза (рис. 5b). Что связано с гораздо большим количеством данных из пула исследований, использующих лабораторную спектроскопию.
Рис. 5.
Сравнение результатов оценки содержания органического углерода почв между спектроскопией в лаборатории и спектроскопией in situ.
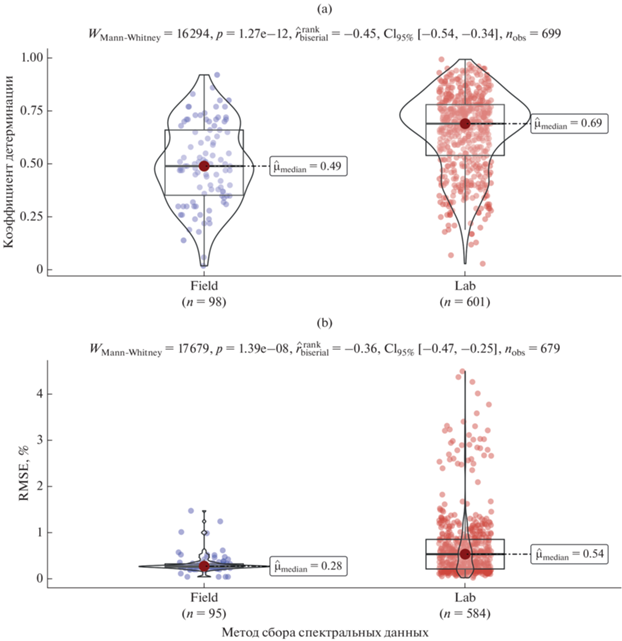
Лучшая производительность лабораторной спектроскопии связана с использованием образцов в виде “идеальной” среды – высушенных, растертых, с предварительным удалением растительного и прочего материала. Подобная пробоподготовка входит в стандарты и протоколы по спектроскопии диффузного рассеяния [14]. Однако это может приводить к увеличению коэффициентов отражения и изменению характера спектральных кривых и выраженности локальных экстремумов [55]. Общими преимуществами лабораторной спектроскопии являются измерения в контролируемых условиях, например, при постоянном источнике освещения, отсутствии атмосферного воздействия.
Спектроскопия in situ экономит дополнительные ресурсы за счет устранения необходимости пробоподготовки образцов. Однако точность прогнозирования страдает от влияния влажности, наличия шероховатости поверхности. Для полевой спектроскопии увеличение влажности почвы вызывает, например, выраженные особенности поглощения при 1400 и 1900 нм, а также снижение общего альбедо [62]. Увеличение влажности почвы также имеет тенденцию маскировать спектральные характеристики компонентов почвы.
Если спектроскопия выступает в качестве базы для дальнейшего картографирования содержания органического углерода почв с помощью данных дистанционного зондирования открытой поверхности почв – необходимо использовать данные инситного спектрометрирования (в идеале подспутникового) для установления зависимостей. Подобные подходы к прогнозированию содержания углерода почв успешно применялись к гиперспектральным спутниковым данным APEX [19] и AsiaFenix [65], а также к мультиспектральным данным Sentinel-2 [16, 18], Landsat [74]. При этом при переходе к данным спутниковой информации модели зачастую теряют в точности (по сравнению с калибровочными моделями на основе данных спектроскопии in situ), что связано с несколькими причинами: искусственные шумы, вызванные конструктивными особенностями датчиков и сенсоров, условия рельефа, атмосферные условия, состояние поверхности почв и другие. Недавние исследования показывают, что добавление в анализ информации о состоянии поверхности позволяет компенсировать негативное воздействие внешних факторов и получить более устойчивые и точные модели [50].
ЗАКЛЮЧЕНИЕ
Vis-NIR спектроскопия диффузного рассеяния представляет хороший вариант менее затратной и быстрой альтернативы традиционным методам анализа свойств почв с приемлемой точностью. По мере развития технологии, совершенствования приборной техники, развития методов анализа данных следует ожидать более убедительных результатов от использования этого подхода в будущем.
Метаанализ с общей выборкой в 709 значений количественных метрик показал, что медианное значение коэффициента детерминации (R2) по всем исследованиям составляет 0.67, ошибки прогноза (RMSE) – 0.48, RPD – 1.99.
Как показал анализ, в настоящее время недостаточно данных для систематического подтверждения точности полевых измерений оценки содержания органического углерода почв с помощью Vis-NIR спектроскопии. В этом направлении необходимы дальнейшие исследования.
Список литературы
Андроников В.Л. О спектральной отражательной способности некоторых почв лесостепи // Изв. АН СССР. Сер. геогр. 1958. № 3. С. 93–97.
Караванова Е.И. Оптические свойства почв и их природа. М.: Изд-во МГУ, 2003. 151 с.
Карманов И.И. Спектральная отражательная способность и цвет почв как показатель их свойств. М.: Колос, 1974. 351 с.
Карманов И.И. Изучение почв по спектральному составу отраженных излучений // Почвоведение. 1970. № 4. С. 34–47.
Кононова М.М. Органическое вещество почвы. Его природа, свойства и методы изучения. М.: Изд-во АН СССР, 1963. 314 с.
Ливеровский Ю.А. Применение аэрометодов при изучении почвенного покрова // Аэрометоды изучения природных ресурсов. М.: Географгиз, 1962. С. 115–129.
Михайлова Н.А., Орлов Д.С. Оптические свойства почв и почвенных компонентов. М.: Наука, 1986. 118 с.
Орлов Д.С., Суханова Н.И., Розанова М.С. Спектральная отражательная способность почв и их компонентов. М.: Изд-во МГУ, 2001. 176 с.
Родионова О.Е., Померанцев А.Л. Хемометрика: достижения и перспективы // Успехи химии. № 4. С. 302–321.
Савин И.Ю., Виндекер Г.В. Некоторые особенности использования оптических свойств почв поверхности для определения их влажности // Почвоведение. 2021. № 7. С. 806–814. https://doi.org/10.31857/S0032180X21070121
Толчельников Ю.С. Оптические свойства ландшафта (применительно к аэросъемке). Л.: Наука, 1974. 252 с.
Barra I., Haefele S.M., Sakrabani R., Kebede F. Soil spectroscopy with the use of chemometrics, machine learning and pre-processing techniques in soil diagnosis: Recent advances – A review // TrAC Trends Anal. Chem. 2021. V. 135. https://doi.org/10.1016/j.trac.2020.116166
Behrens T., Viscarra Rossel R.A., Ramirez-Lopez L., Baumann P. Soil spectroscopy with the Gaussian pyramid scale space // Geoderma. 2022. V. 426. https://doi.org/10.1016/j.geoderma.2022.116095
Ben Dor E., Ong C., Lau I.C. Reflectance measurements of soils in the laboratory: Standards and protocols // Geoderma. 2015. V. 245–246. P. 112–124. https://doi.org/10.1016/j.geoderma.2015.01.002
Buddenbaum H., Steffens M. The effects of spectral pretreatments on chemometric analyses of soil profiles using laboratory imaging spectroscopy // Appl. Environ. Soil Sci. 2012. V. 2012. P. 1–12. https://doi.org/10.1155/2012/274903
Castaldi F. Sentinel-2 and Landsat-8 Multi-Temporal Series to Estimate Topsoil Properties on Croplands // Remote Sens. 2021. V. 13. https://doi.org/10.3390/rs13173345
Castaldi F., Chabrillat S., Chartin C., Genot V., Jones A.R., van Wesemael B. Estimation of soil organic carbon in arable soil in Belgium and Luxembourg with the LUCAS topsoil database // Eur. J. Soil Sci. 2018b. V. 69. P. 592–603. https://doi.org/10.1111/ejss.12553
Castaldi F., Chabrillat S., Don A., van Wesemael B. Soil organic carbon mapping using LUCAS topsoil database and sentinel-2 data: an approach to reduce soil moisture and crop residue effects // Remote Sens. 2019. V. 11. https://doi.org/10.3390/rs11182121
Castaldi F., Chabrillat S., Jones A., Vreys K., Bomans B., van Wesemael B. Soil organic carbon estimation in croplands by hyperspectral remote APEX data using the LUCAS topsoil database // Remote Sens. 2018. V. 10. https://doi.org/10.3390/rs10020153
Chakraborty S., Li B., Weindorf D.C., Morgan C.L.S. External parameter orthogonalisation of Eastern European VisNIR-DRS soil spectra // Geoderma. 2019. V. 337. P. 65–75. https://doi.org/10.1016/j.geoderma.2018.09.015
Chinilin A.V., Savin I.Yu. Comparison of the effectiveness of various ways of preprocessing spectrometric data in order to predict the concentration of organic soil carbon // J. Opt. Technol. 2018. V. 85. P. 789–795. https://doi.org/10.1364/JOT.85.000789
Coblinski J.A., Giasson É., Demattê J.A.M., Dotto A.C., Costa J.J.F., Vašát R. Prediction of soil texture classes through different wavelength regions of reflectance spectroscopy at various soil depths // Catena. 2020. V. 89. https://doi.org/10.1016/j.catena.2020.104485
Condit H.R. The spectral reflectance of American soils // Photogramm. Eng. 1970. V. 36. P. 955–966.
Dotto A.C., Dalmolin R.S.D., ten Caten A., Grunwald S. A systematic study on the application of scatter-corrective and spectral-derivative preprocessing for multivariate prediction of soil organic carbon by Vis-NIR spectra // Geoderma. 2018. V. 314. P. 262–274. https://doi.org/10.1016/j.geoderma.2017.11.006
Gholizadeh A., Coblinski J.A., Saberioon M., Ben-Dor E., Drábek O., Demattê J.A.M., Borůvka L. vis–NIR and XRF Data Fusion and Feature Selection to Estimate Potentially Toxic Elements in Soil // Sensors. 2021. V. 21. https://doi.org/10.3390/s21072386
Gholizadeh A., Viscarra Rossel R.A., Saberioon M., Borůvka L., Kratina J., Pavlů L. National-scale spectroscopic assessment of soil organic carbon in forests of the Czech Republic // Geoderma. 2021b. V. 385. https://doi.org/10.1016/j.geoderma.2020.114832
Gozukara G., Akça E., Dengiz O., Kapur S., Adak A. Soil particle size prediction using Vis-NIR and pXRF spectra in a semiarid agricultural ecosystem in Central Anatolia of Türkiye // Catena. 2022. V. 217. https://doi.org/10.1016/j.catena.2022.106514
Gozukara G., Hartemink A.E., Zhang Y. Soil Catena characterization using pXRF and Vis-NIR spectroscopy in Northwest Turkey // Eurasian Soil Sci. 2021. V. 54. P. 1–15. https://doi.org/10.1134/S1064229322030061
Hong Y., Chen Y., Chen S., Shen R., Guo L., Liu Y., Mounem Mouazen A. Improving spectral estimation of soil inorganic carbon in urban and suburban areas by coupling continuous wavelet transform with geographical stratification // Geoderma. 2023. V. 430. https://doi.org/10.1016/j.geoderma.2022.116284
Igne B., Reeves J.B., McCarty G., Hively W.D., Lund E., Hurburgh C.R. Evaluation of spectral pretreatments, partial least squares, least squares support vector machines and locally weighted regression for quantitative spectroscopic analysis of soils // J. Near Infrared Spectrosc. 2010. V. 18. P. 167–176. https://doi.org/10.1255/jnirs.883
Khayamim F., Wetterlind J., Khademi H., Robertson A.H.J., Cano A.F., Stenberg B. Using visible and near infrared spectroscopy to estimate carbonates and gypsum in soils in arid and subhumid regions of Isfahan, Iran // J. Near Infrared Spectrosc. 2015. V. 23. P. 155–165. https://doi.org/10.1255/jnirs.1157
Knadel M., Castaldi F., Barbetti R., Ben-Dor E., Gholizadeh A., Lorenzetti R. Mathematical techniques to remove moisture effects from visible–near-infrared–shortwave-infrared soil spectra – review // Appl. Spectrosc. Rev. 2022. P. 1–34. https://doi.org/10.1080/05704928.2022.2128365
Knox N.M.M., Grunwald S., McDowell M.L.L., Bruland G.L.L., Myers D.B.B., Harris W.G.G. Modelling soil carbon fractions with visible near-infrared (VNIR) and mid-infrared (MIR) spectroscopy // Geoderma. 2015. V. 239–240. P. 229–239. https://doi.org/10.1016/j.geoderma.2014.10.019
Kuhn M., Johnson K. Applied Predictive Modeling. N.Y.: Springer. 2013. 600 p. https://doi.org/10.1007/978-1-4614-6849-3
Li S., Viscarra Rossel R.A., Webster R. The cost-effectiveness of reflectance spectroscopy for estimating soil organic carbon // Eur. J. Soil Sci. 2022. V. 73. https://doi.org/10.1111/ejss.13202
Liu Y., Pan X.-Z.X., Wang C., Li Y., Shi R. Predicting soil salinity with Vis–NIR spectra after removing the effects of soil moisture using external parameter orthogonalization // PLoS One. 2015. V. 10. https://doi.org/10.1371/journal.pone.0140688
Mallat S.G. A theory for multiresolution signal decomposition: the wavelet representation // IEEE Trans. Pattern Anal. Mach. Intell. 1989. V. 11. P. 674–693. https://doi.org/10.1109/34.192463
Martens H., Næs T. Multivariate calibration // Chemometrics. Netherlands, Dordrecht: Springer. 1984. P. 147–156. https://doi.org/10.1007/978-94-017-1026-8_5
McBride M.B. Estimating soil chemical properties by diffuse reflectance spectroscopy: Promise versus reality // Eur. J. Soil Sci. 2022. V. 73. https://doi.org/10.1111/ejss.13192
Mendes W. de S., Demattê J.A.M., Bonfatti B.R., Resende M.E.B., Campos L.R., da Costa A.C.S. A novel framework to estimate soil mineralogy using soil spectroscopy // Appl. Geochem. 2021. V. 127. https://doi.org/10.1016/j.apgeochem.2021.104909
Minasny B., McBratney A.B. Digital soil mapping: A brief history and some lessons // Geoderma. 2016. V. 264. P. 301–311. https://doi.org/10.1016/j.geoderma.2015.07.017
Molnar C. Interpretable Machine Learning: A Guide For Making Black Box Models Explainable. 2022. https://christophm.github.io/interpretable-ml-book/
Morellos A., Pantazi X.-E., Moshou D., Alexandridis T., Whetton R., Tziotzios G., Wiebensohn J. Machine learning based prediction of soil total nitrogen, organic carbon and moisture content by using VIS-NIR spectroscopy // Biosyst. Eng. 2016. V. 152. P. 104–116. https://doi.org/10.1016/j.biosystemseng.2016.04.018
Moura-Bueno J.M., Dalmolin R.S.D., ten Caten A., Dotto A.C., Demattê J.A.M. Stratification of a local VIS-NIR-SWIR spectral library by homogeneity criteria yields more accurate soil organic carbon predictions // Geoderma. 2019. V. 337. P. 565–581. https://doi.org/10.1016/j.geoderma.2018.10.015
Muñoz J.D., Kravchenko A. Soil carbon mapping using on-the-go near infrared spectroscopy, topography and aerial photographs // Geoderma. 2011. V. 166. P. 102–110. https://doi.org/10.1016/j.geoderma.2011.07.017
Ng W., Malone B., Minasny B., Jeon S. Near and mid infrared soil spectroscopy // Reference Module in Earth Systems and Environmental Sciences. Elsevier, 2022. https://doi.org/10.1016/B978-0-12-822974-3.00022-7
Ng W., Minasny B., McBratney A. Convolutional neural network for soil microplastic contamination screening using infrared spectroscopy // Sci. Total Environ. 2020. V. 702. https://doi.org/10.1016/j.scitotenv.2019.134723
Patil I. Visualizations with statistical details: The “ggstatsplot” approach // J. Open Source Softw. 2021. V. 6. https://doi.org/10.21105/joss.03167
Poppiel R.R., Paiva A.F. da S., Demattê J.A.M. Bridging the gap between soil spectroscopy and traditional laboratory: Insights for routine implementation // Geoderma. 2022. V. 425. https://doi.org/10.1016/j.geoderma.2022.116029
Prudnikova E., Savin I.Yu. Some Peculiarities of Arable Soil Organic Matter Detection Using Optical Remote Sensing Data // Remote Sens. 2021. V. 13. https://doi.org/10.3390/rs13122313
Ramos P.V., Inda A.V., Barrón V., Siqueira D.S., Marques Júnior J., Teixeira D.D.B. Color in subtropical brazilian soils as determined with a Munsell chart and by diffuse reflectance spectroscopy // Catena. 2020. V. 193. https://doi.org/10.1016/j.catena.2020.104609
Rehman H.U., Knadel M., Jonge L.W., Moldrup P., Greve M.H., Arthur E. Comparison of cation exchange capacity estimated from Vis–NIR spectral reflectance data and a pedotransfer function // Vadose Zo. J. 2019. V. 18. P. 1–8. https://doi.org/10.2136/vzj2018.10.0192
Roudier P., Hedley C.B., Lobsey C.R., Viscarra Rossel R.A., Leroux C. Evaluation of two methods to eliminate the effect of water from soil vis–NIR spectra for predictions of organic carbon // Geoderma. 2017. V. 296. P. 98–107. https://doi.org/10.1016/j.geoderma.2017.02.014
dos Santos U.J., Demattê J.A.M., Menezes R.S.C., Dotto A.C., Guimarães C.C.B., Alves B.J.R., Primo D.C. Predicting carbon and nitrogen by visible near-infrared (Vis-NIR) and mid-infrared (MIR) spectroscopy in soils of Northeast Brazil // Geoderma Reg. 2020. V. 23. https://doi.org/10.1016/j.geodrs.2020.e00333
Savin I.Y., Shishkin M.A., Sharychev D.V. Peculiarities of spectral reflectance of fractions with sizes from 20 to 5,000 microns in soil samples // Dokuchaev Soil Bull. 2022. V. 112. P. 24–47. https://doi.org/10.19047/0136-1694-2022-112-24-47
Savitzky A., Golay M.J.E. Smoothing and Differentiation of Data by Simplified Least Squares Procedures // Anal. Chem. 1964. V. 36. P. 1627–1639. https://doi.org/10.1021/ac60214a047
Slaughter D.C., Pelletier M.G., Upadhyaya S.K. Sensing soil moisture using NIR spectroscopy // Appl. Eng. Agric. 2001. V. 17. https://doi.org/10.13031/2013.5449
Song Y., Shen Z., Wu P., Viscarra Rossel R.A. Wavelet geographically weighted regression for spectroscopic modelling of soil properties // Sci. Rep. 2021. V. 11. https://doi.org/10.1038/s41598-021-96772-z
Sorenson P.T., Small C., Tappert M.C., Quideau S.A., Drozdowski B., Underwood A., Janz A. Monitoring organic carbon, total nitrogen, and pH for reclaimed soils using field reflectance spectroscopy // Can. J. Soil Sci. 2017. V. 97. P. 241–248. https://doi.org/10.1139/cjss-2016-0116
Soriano-Disla J.M.J.M., Janik L.J., Viscarra Rossel R.A., MacDonald L.M., McLaughlin M.J. The Performance of visible, near-, and mid-infrared reflectance spectroscopy for prediction of soil physical, chemical, and biological properties // Appl. Spectrosc. Rev. 2014. V. 49. P. 139–186. https://doi.org/10.1080/05704928.2013.811081
Steffens M., Zeh L., Rogge D.M., Buddenbaum H. Quantitative mapping and spectroscopic characterization of particulate organic matter fractions in soil profiles with imaging VisNIR spectroscopy // Sci. Rep. 2021. V. 11. https://doi.org/10.1038/s41598-021-95298-8
Stenberg B., Viscarra Rossel R.A., Mouazen A.M., Wetterlind J. Visible and near infrared spectroscopy in soil science // Adv. in Agr. 2010. V. 107. P. 163–215. https://doi.org/10.1016/S0065-2113(10)07005-7
Stevens A., Nocita M., Tóth G., Montanarella L., van Wesemael B. Prediction of soil organic carbon at the european scale by visible and near infrared reflectance spectroscopy // PLoS One. 2013. V. 8. https://doi.org/10.1371/journal.pone.0066409
Stevens A., Ramirez-Lopez L. An introduction to the “prospectr” package. R package version 0.2.6. https://cran.r-project.org/package=prospectr
Tziolas N., Tsakiridis N., Ogen Y., Kalopesa E., Ben-Dor E., Theocharis J., Zalidis G. An integrated methodology using open soil spectral libraries and Earth Observation data for soil organic carbon estimations in support of soil-related SDGs // Remote Sens. Environ. 2020. V. 244. https://doi.org/10.1016/j.rse.2020.111793
Viscarra Rossel R.A., Behrens T., Ben-Dor E., Chabrillat S., Demattê J.A.M., Ge Y., Gomez C. Diffuse reflectance spectroscopy for estimating soil properties: A technology for the 21st century // Eur. J. Soil Sci. 2022. V. 73. https://doi.org/10.1111/ejss.13271
Viscarra Rossel R.A., Bui E.N., De Caritat P., McKenzie N.J. Mapping iron oxides and the color of Australian soil using visible-near-infrared reflectance spectra // J. Geophys. Res. Earth Surf. 2010. V. 115. https://doi.org/10.1029/2009JF001645
Viscarra Rossel R.A., Cattle S.R., Ortega A., Fouad Y. In situ measurements of soil colour, mineral composition and clay content by vis–NIR spectroscopy // Geoderma. 2009. V. 150. P. 253–266. https://doi.org/10.1016/j.geoderma.2009.01.025
Viscarra Rossel R.A., Hicks W.S. Soil organic carbon and its fractions estimated by visible-near infrared transfer functions // Eur. J. Soil Sci. 2015. V. 66. P. 438–450. https://doi.org/10.1111/ejss.12237
Viscarra Rossel R.A., Jeon Y.S., Odeh I.O.A., McBratney A.B. Using a legacy soil sample to develop a mid-IR spectral library // Soil Res. 2008. V. 46. https://doi.org/10.1071/SR07099
Viscarra Rossel R.A., McBratney A.B., Minasny B. Proximal Soil Sensing. N.Y.: Springer, 2010. 468 p. https://doi.org/10.1007/978-90-481-8859-8
Wadoux A.M.J.-C., Malone B., Minasny B., Fajardo M., McBratney A.B. Soil Spectral Inference with R. N.Y.: Springer, 2021. 247 p. https://doi.org/10.1007/978-3-030-64896-1
Wan M., Hu W., Qu M., Li W., Zhang C., Kang J., Hong Y. Rapid estimation of soil cation exchange capacity through sensor data fusion of portable XRF spectrometry and Vis-NIR spectroscopy // Geoderma. 2020. V. 363. https://doi.org/10.1016/j.geoderma.2019.114163
Wang X., Zhang Y., Atkinson P.M., Yao H. Predicting soil organic carbon content in Spain by combining Landsat TM and ALOS PALSAR images // Int. J. Appl. Earth Obs. Geoinf. 2020. V. 92. https://doi.org/10.1016/j.jag.2020.102182
Wang Y., Huang T., Liu J., Lin Z., Li S., Wang R., Ge Y. Soil pH value, organic matter and macronutrients contents prediction using optical diffuse reflectance spectroscopy // Comput. Electron. Agric. 2015. V. 111. P. 69–77. https://doi.org/10.1016/j.compag.2014.11.019
Wetterlind J., Viscarra Rossel R.A., Steffens M. Diffuse reflectance spectroscopy characterises the functional chemistry of soil organic carbon in agricultural soils // Eur. J. Soil Sci. 2022. V. 73. https://doi.org/10.1111/ejss.13263
Xiaobo Z., Jiewen Z., Povey M.J.W., Holmes M., Hanpin M. Variables selection methods in near-infrared spectroscopy // Anal. Chim. Acta. 2010. V. 667. P. 14–32. https://doi.org/10.1016/j.aca.2010.03.048
Xu S., Zhao Y., Wang M., Shi X. Quantification of Different Forms of Iron from Intact Soil Cores of Paddy Fields with Vis-NIR Spectroscopy // Soil Sci. Soc. Am. J. 2018. V. 82. P. 1497–1511. https://doi.org/10.2136/sssaj2018.01.0014
Yang Y., Shen Z., Bissett A., Viscarra Rossel R.A. Estimating soil fungal abundance and diversity at a macroecological scale with deep learning spectrotransfer functions // Soil. 2022. V. 8. P. 223–235. https://doi.org/10.5194/soil-8-223-2022
Yang Y., Viscarra Rossel R.A., Li S., Bissett A., Lee J., Shi Z., Behrens T., Court L. Soil bacterial abundance and diversity better explained and predicted with spectro-transfer functions // Soil Biol. Biochem. 2019. V. 129. P. 29–38. https://doi.org/10.1016/j.soilbio.2018.11.005
Zhong L., Guo X., Xu Z., Ding M. Soil properties: Their prediction and feature extraction from the LUCAS spectral library using deep convolutional neural networks // Geoderma. 2021. V. 402. https://doi.org/10.1016/j.geoderma.2021.115366
Zhou Y., Chen S., Hu B., Ji W., Li S., Hong Y., Xu H. Global soil salinity prediction by open soil Vis-NIR spectral library // Remote Sens. 2022. V. 14. https://doi.org/10.3390/rs14215627
Ziechmann W. Spectroscopic investigations of lignin, humic substances and peat // Geochim. Cosmochim. Acta. 1964. V. 28. P. 1555–1566. https://doi.org/10.1016/0016-7037(64)90006-7
Дополнительные материалы отсутствуют.